To Be High-Risk, or Not To Be—Semantic Specifications and Implications of the AI Act’s High-Risk AI Applications and Harmonised Standards
Conference on Fairness, Accountability, and Transparency (FAccT)
✍ Delaram Golpayegani* , Harshvardhan J. Pandit , Dave Lewis
Description: This article analyses the core concepts in EU Act's high-risk categorisation, and presents an open vocabulary to model AI risk. It also explores the implications of standardisation activities in connection with AI Act.
published version 🔓open-access archives: DORAS , harshp.com , TARA , zenodo
📦resources: To Be High-Risk, or Not To Be—Semantic Specifications and Implications of the AI Act’s High-Risk AI Applications and Harmonised Standards , specification
Abstract The EU's proposed AI Act sets out a risk-based regulatory framework to govern the potential harms emanating from use of AI systems. Within the AI Act's hierarchy of risks, the AI systems that are likely to incur \emph{``high-risk''} to health, safety, and fundamental rights are subject to the majority of the Act's provisions. To include uses of AI where fundamental rights are at stake, Annex III of the Act provides a list of applications wherein the conditions that shape high-risk AI are described. For high-risk AI systems, the AI Act places obligations on providers and users regarding use of AI systems and keeping appropriate documentation through the use of harmonised standards. In this paper, we analyse the clauses defining the criteria for high-risk AI in Annex III to simplify identification of potential high-risk uses of AI by making explicit the ``core concepts'' whose combination makes them high-risk.
We use these core concepts to develop an open vocabulary for AI risks (VAIR) to represent and assist with AI risk assessments in a form that supports automation and integration. VAIR is intended to assist with identification and documentation of risks by providing a common vocabulary that facilitates knowledge sharing and interoperability between actors in the AI value chain. Given that the AI Act relies on harmonised standards for much of its compliance and enforcement regarding high-risk AI systems, we explore the implications of current international standardisation activities undertaken by ISO and emphasise the necessity of better risk and impact knowledge bases such as VAIR that can be integrated with audits and investigations to simplify the AI Act's application.
Introduction
The EU AI Act [1], the first proposed legal regime for development and use of AI systems, sets out a risk-based approach and proposes binding requirements for those who provide and use “high-risk” AI systems that are likely to cause serious harms to health, safety, or fundamental rights of individuals. The AI Act applies the high-risk concept to AI systems used as products and safety components of products already covered by EU harmonisation legislation. Further, it defines specific uses of AI as being high-risk, with a list provided in Annex III and provisions for the European Commission to modify the list in future amendments. With any update to the high-risk list, AI providers, by whom the majority of compliance obligations should be satisfied, need to undertake an assessment to find out if their systems fall into the newly introduced areas.
Considering the EU’s global influence on technology-related rulemaking, which has already manifested in the data protection area with the enforcement of the General Data Protection Regulation (GDPR) GDPR?, soon similar AI regulations are expected to be developed by governments worldwide. Reaching a global consensus on high-risk areas as defined by the AI Act is highly unlikely, which means there will likely be multiple diverging risk-based classifications in different jurisdictions. This represents legal uncertainties for stakeholders as an AI system could potentially be or not be high-risk based on the geopolitical contexts it is used in. For example, social credit scoring systems are banned in the EU (AI Act, Art. 5(1)(c)) while an implementation of this is being used in China [2]. Following from these, stakeholders thus face a challenge in how to structure, document, and share information in the context of their AI systems or components such that this information assists with fulfilling different regulatory requirements without impeding rapid progress in global markets.
Under the AI Act, high-risk AI systems have specific obligations regarding identification, management, and documentation of risks. To support implementation of such high-level legal requirements, the Act relies on harmonised standards created by European standardisation organisations. However, in reality, the Act and its effectiveness face the following issues at present:
Lack of clarity and guidelines regarding determination of high-risk uses of AI listed in Annex III;
Lack of standardised methods for representing and investigating risk management in use-cases involving AI;
Lack of guidance on how risk documentation and knowledge should be provided between actors, especially where providers and users are not developers of an AI system or its components.
To address these challenges, we analysed the AI Act, with a focus on Annex III, to create a simplified and structured framework that not only assists with discovering whether an AI use-case falls under the AI Act’s high-risk categorisation, but also helps with identification of relevant risks and their potential impacts. Finally, we analyse the state of the standards within ISO and CEN-CENELEC to understand the relevance of published and under-development AI standards to the AI Act’s high-risk AI requirements. In this research, we provide the following contributions:
A simplified and structured framework for identification of potential high-risk uses of AI as per Annex III (Section 3);
An open and interoperable vocabulary for representing, documenting, and sharing AI risk information and best practices (Section 4);
An analysis of the scope of standardisation activities within ISO and CEN-CENELEC in regard to the AI Act’s provisions concerning high-risk AI (Section 5).
Background and Related Work
The AI Act
Legislation Development Process: Following the ordinary legislative process1, the AI Act was first proposed by the European Commission in April 20212 as a binding instrument to guard individuals in the European Union against AI-related harms. The proposal has to be approved by both the European Parliament and the Council of the European Union to be passed as EU legislation. At the end of its term in June 2022, the French presidency of the Council published a consolidated version3. The Council’s common position, the latest draft of the Act at the time of writing, was issued in November 2022 by the Czech presidency. During the first reading of the Act in the European parliament, more than 3000 amendments were tabled by the responsible committees, namely the Committee on the Internal Market and the Committees on Consumer Protection and Civil Liberties, Justice and Home Affairs. Finalisation of the Parliament’s position, which is expected in the first semester of 2023, will allow entering the trilogue phase, whereby the Commission, Parliament, and Council negotiate the AI Act behind closed doors to reach an agreement on the final text. 12 days after the publication of the Act in the Official Journal of the European Union, it will come into force (Art. 85(1)) and 36 months after, it will be applied (Art. 85(2)). In this paper, we adopt the Council’s common position on the AI Act.
Structure and Content: The AI Act’s key feature is its risk-based structure where different legal regimes are established for governing AI systems according to their potential detrimental impacts on health, safety, and fundamental rights. These legal regimes cover four clusters of AI systems with (i) unacceptable (severe), (ii) high, (iii) limited, and (iv) minimal risks. Rather than providing a comprehensive overview of the Act’s content, we focus on the high-risk regime (described in Title III), which follows the new legislative framework (NLF)—a common EU product-related legal framework adopted in 2008. According to Art. 6, an AI system classifies as high-risk if it is: (1) a product which requires third-party conformity assessment under at least one of the Union harmonisation legislations listed in Annex II; (2) used as a safety component of a product mentioned in the preceding point; or (3) used in the use-cases described in Annex III. Chapter 2 of Title III prescribes the essential requirements that a high-risk AI system should fulfil, including having a risk management system in place (Art. 9) and being accompanied by technical documentation (Art. 11). Legal provisions applied to high-risk AI providers, users, and other related actors such as importers and distributors are described in Chapter 3. Following the NLF, the Act introduces harmonised standards as instruments for providing detailed technical solutions for compliance with essential requirements. Owing to the presumption of conformity (Art. 40), AI providers can achieve compliance with the requirements through conformance to harmonised standards, without undergoing the costly and time-consuming process of requirements interpretation [3].
Views on the AI Act’s High-Risk AI Areas
While there have been several comments and opinions published regarding the AI Act, we focus on the concerns raised regarding high-risk areas. In one of the first and highly-cited analyses of the Act, Veale and Borgesius [3] bring up the insufficiency of Annex III high-risk areas in addressing applications where fundamental rights are at risk. De Cooman [4] argues the AI Act’s deficiency in addressing the full range of risks associated with AI systems by referring to the potential harms of non-high-risk AI, i.e. AI systems with limited or minimal risk. The author also highlights the importance of culture and social tolerance in determining harmful applications of AI. In agreement with the aforementioned views, Ebers et al. [5] reflect on the areas where the AI Act’s high-risk list falls short of: (i) the missing high-risk contexts of AI use, e.g. use of AI for housing purposes, and (ii) the ignored harms of AI to groups which in turn affect individuals, e.g. discrimination caused by AI systems used for predictive policing. The authors also suggest expanding the AI Act’s risk hierarchy to a more detailed and granular risk categorisation. We take up this suggestion and propose a vocabulary for AI risks in Section 4.
According to AI Act’s Art.7, the Commission is granted the legislative power to amend the list of high-risk AI systems in Annex III and thereby introduce new criteria for high-risk AI based on perceived harms. However, this ability is restricted to only those areas already mentioned in Annex III. This limitation in adding new areas is criticised in [5] and [6], where the authors highlight the necessity of extending the high-risk areas.
Taxonomies for Describing AI Systems and Their Risks
There are multiple generic taxonomies for describing harmful applications of AI. The AI, algorithmic, and automation incidents and controversies (AIAAIC) repository4 is an open-access dataset of more than 900 AI incidents covered by the media. The AIAAIC taxonomy provides a set of concepts for incident annotation, including categories of sectors, technologies, purposes, and impacts of AI on individuals, society, environment, and providers. The Partnership on AI’s AI incident database (AIID) [7] is a crowd-sourced database of 24000 incidents. The creation of the taxonomy followed a bottom-up approach where the taxonomy is populated through incident annotation [8]. AITopics5 is the AAAI’s (Association for the Advancement of Artificial Intelligence) corpus of AI-related news stories, research articles, conferences, and journals. The scope of AITopics is not limited to AI incidents and therefore it indexes all types of AI-related news articles as well as scientific papers. Discovery, categorisation (determining the main focus), and summarisation of AI news featured in AITopics are automated [9]. The OECD’s framework for classification of AI systems is a tool for assessing potential risks and benefits of AI use-cases by considering five high-level dimensions: people & planet, economic context, data & input, AI model, and task & output. The framework incorporates taxonomies for its risk assessment criteria. Developing a common framework for reporting AI incidents is on the OECD’s agenda for future work [10]. The AI risk ontology (AIRO) [11] is an ontology for modelling AI systems and their associated risks. AIRO, which is built upon the AI Act and ISO 31000 family of risk management standards, includes instances of AI and risk concepts organised in a hierarchical manner. Table [tab:ai risk taxonomy] provides an overview of the taxonomies provided by the above-mentioned work for describing AI systems and their associated risks.
In addition to generic AI taxonomies, an active area of research is identification of taxonomies for risks associated with specific AI techniques or specific types of risks e.g. bias. Examples of these are: Weidinger et al.’s taxonomy of ethical and social risks of language models [12], the open loop’s taxonomy of potential harms associated with machine learning applications and automated decision-making systems [13], NIST’s taxonomy of adversarial machine learning [14] and categories of AI Bias [15], Steimers and Schneider’s work on creating a taxonomy of risk sources that impact AI trustworthiness [16], and Roselli et al.’s work on classification of AI bias [17].
AIAAIC | AIID | AITopics | OECD taxonomy | AIRO | |
---|---|---|---|---|---|
Main resource | News articles | News articles | Web resources | Related research | AI Act, ISO 31000 |
Development methodology | Bottom-up, manual discovery & annotation | Bottom-up, manual discovery & annotation | Automated discovery & annotation | Unknown | Top-down |
AI taxonomies | Technology | AI functions AI techniques Developer | Technology | Application area AI system task | AI technique |
Use of AI taxonomies | Sector Purpose | Sector of deployment Nature of end-users | Industry | User Industrial sector Business function | Purpose Stakeholder |
Risk and impact taxonomies | Transparency issue External impact Internal impact | AI harm Materialisation of harm Sectors affected | — | Impacted stakeholders Impact Redress | Risk source Consequence Impact Control |
Analysis and Semantification of the AI Act’s High-Risk AI Use-Cases
As shown in the previous section, taxonomies of AI risks are predominantly built through annotation of AI incidents. The information captured from incidents enables reverse causal inference to identify why an AI system caused harm (causes of effects). As the AI Act serves a precautionary role, it articulates what situations are likely to pose high risk to health, safety, and fundamental rights ; and lays down requirements to avoid incidents that are likely to result in harmful impacts from happening. Among the three main conditions for high-risk AI systems (discussed in Section 2.1), the uses of AI systems described in Annex III primarily refer to situations where fundamental rights are at stake while the main concerns with most of the systems that fall under the already regulated domains, listed in Annex II, are related to health and safety. To assist with identification of high-risk AI systems, we provide a structured and simplified framework by addressing the following practical aspects:
What information is needed to make a decision about whether an application of AI is high-risk as per Annex III?
When should the evaluation re-assessed?
Who is responsible for making the decision, particularly in the case of general purpose AI?
Requirements and Semantic Specifications for Determining High-Risk AI
Annex III represents high-risk uses of AI under 8 areas by providing a brief description of the situations that are likely to harm individuals. For example, under the area of migration, asylum and border control management (Annex III, pt. 7) one of the AI applications qualified as high-risk is described as follows: “AI systems intended to be used by competent public authorities or on their behalf to assess a risk, including a security risk, a risk of irregular immigration, or a health risk, posed by a natural person who intends to enter or has entered into the territory of a Member State” (Annex III, pt. 7(b)).
High-Risk AI Criteria
Inspired by the GDPR’s criteria for determining the necessity of conducting a Data Protection Impact Assessment (DPIA) (GDPR, Art. 35(3)), through an in-depth analysis of the descriptions of high-risk AI use-cases, we identified the following 5 concepts, which are expressed in various combinations by Annex III:
In which domain is the AI system used?
What is the purpose of the AI system?
What is the capability of the AI system?
Who is the user of the AI system?
Who is the AI subject?
In the above-mentioned questions, domain represents the area or sector the AI system is intended to be used in. The AI Act defines intended purpose as “the use for which an AI system is intended by the provider, including the specific context and conditions of use...” (Art. 3(12)); however to avoid complexities regarding context and conditions of use, we describe purpose as an objective that is intended to be accomplished by using an AI system. The AI system’s capability enables realisation of its purpose and reflects the technological capability; for example biometric identification is the capability used towards achieving the purpose of remote identification of people. AI user, as defined in Art. 3(4), is “any natural or legal person, including a public authority, agency or other body, under whose authority the system is used”. AI subject refers to the person subjected to the use of AI; a passenger entering a territory is an example of an AI subject in an AI system used for assessing the risk of irregular immigration.
High-Risk AI Conditions
To specify the conditions where use of an AI system is classified as high-risk, we determined values of the identified concepts by answering the 5 questions for each clause in Annex III. Combinations of values, which can be treated as rules for high-risk uses, for Annex III’s high-risk applications are represented in Figure [fig:conditions]. If an AI system meets at least one of the conditions, it is considered as high-risk unless (i) its provider demonstrates that “the output of the system purely accessory in respect of the relevant action or decision to be taken and is not therefore likely to lead to a significant risk to the health, safety or fundamental rights.” (Art. 6 (3)), or (ii) it is put into service by a small-scale provider in the public or private sector for their own use to assess creditworthiness, determine credit score, health/life insurance risk assessment, or health/life insurance pricing (Annex III, pt. 5(a) and 5(b)).
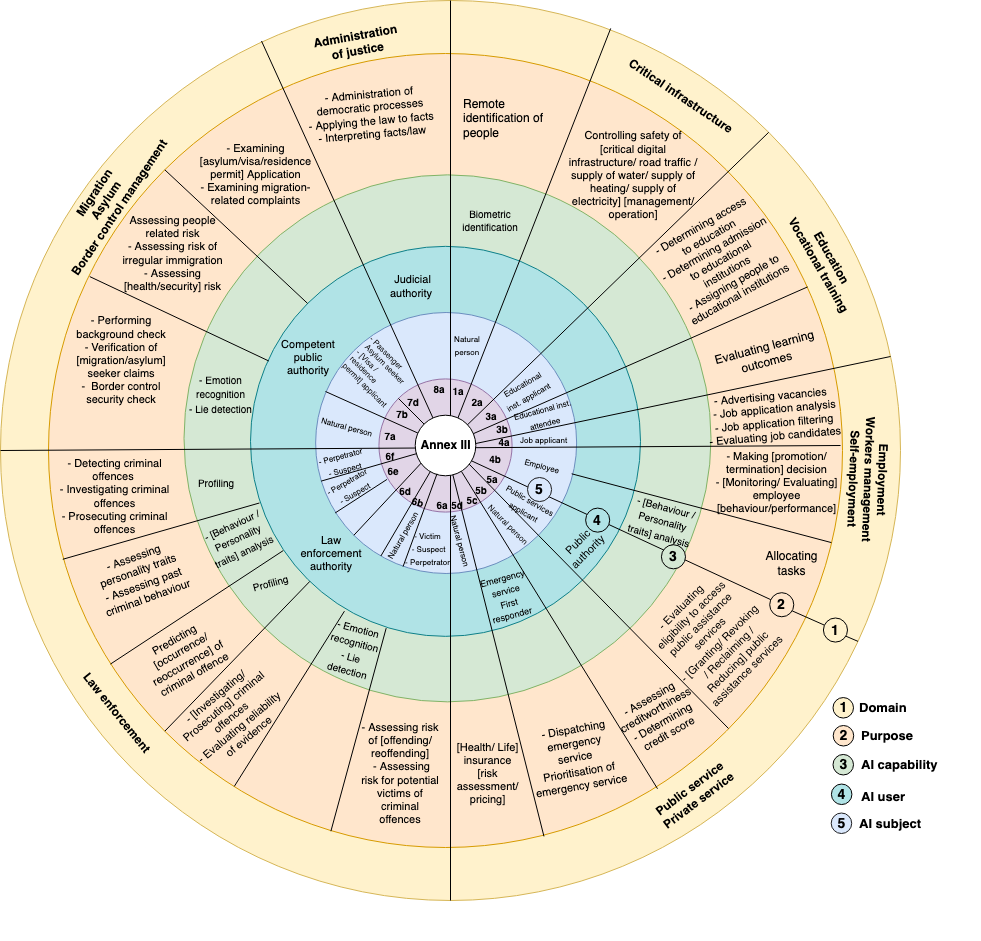
An AI system determined as high-risk should fulfil the requirements recited in Title III Chapter 2, such as having a risk management system operationalised and documented (Art. 9), being accompanied by technical documentation whose content is subject to scrutiny in regard to conformity assessment (Art. 11), and demonstrating appropriate levels of accuracy, robustness and cybersecurity (Art. 15). Ensuring that such a system fulfils the high-risk AI requirements is the obligation of its provider or any actor described in Art. 23a (Art. 16(a)).
Semantic Specifications
We leverage semantic web technologies to provide a standardised way for representing, documenting, and sharing the 5 concepts, to enable automation in making the decision regarding whether or not a particular use of an AI system is qualified as high-risk, and to facilitate investigation and auditing of risk management. In semantic modelling of the concepts, we reused concepts and relations shown in Figure 1 from AIRO. Providing a semantic representation of an AI use-case and semantification of high-risk rules require a vocabulary that represents instances of concepts in a hierarchical manner, e.g. different types of purposes for which AI might be used. To satisfy this requirement, we created a vocabulary for AI risks (see Section 4).
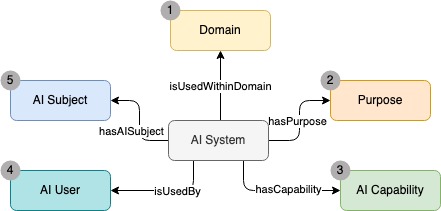
To automate reasoning, we define high-risk rules as target sets using the shapes constraint language (SHACL)6. Based on this, we developed a tool to assist in determining high-risk uses of AI (Figure 2). The tool asks the 5 questions, mentioned earlier, and provides a list of instances from which the user can select a value. Based on the user’s input, an RDF graph that describes the system in a machine-readable format is generated and then the graph is validated against the SHACL shapes to determine if conditions for high-risk AI are met. The output of the current version of the tool includes the result of the assessment (high-risk or not high-risk) and an assessment report. The tool is limited in identification of prohibited AI systems, therefore classification of the system into the prohibited category on the basis of Art. 5 conditions should be ruled out before using the tool. Future enhancements include providing suggestions and guidelines for different stakeholders regarding the next steps, for example providing information about legal requirements, relevant standards, and the additional details required to be maintained for conformity assessment.
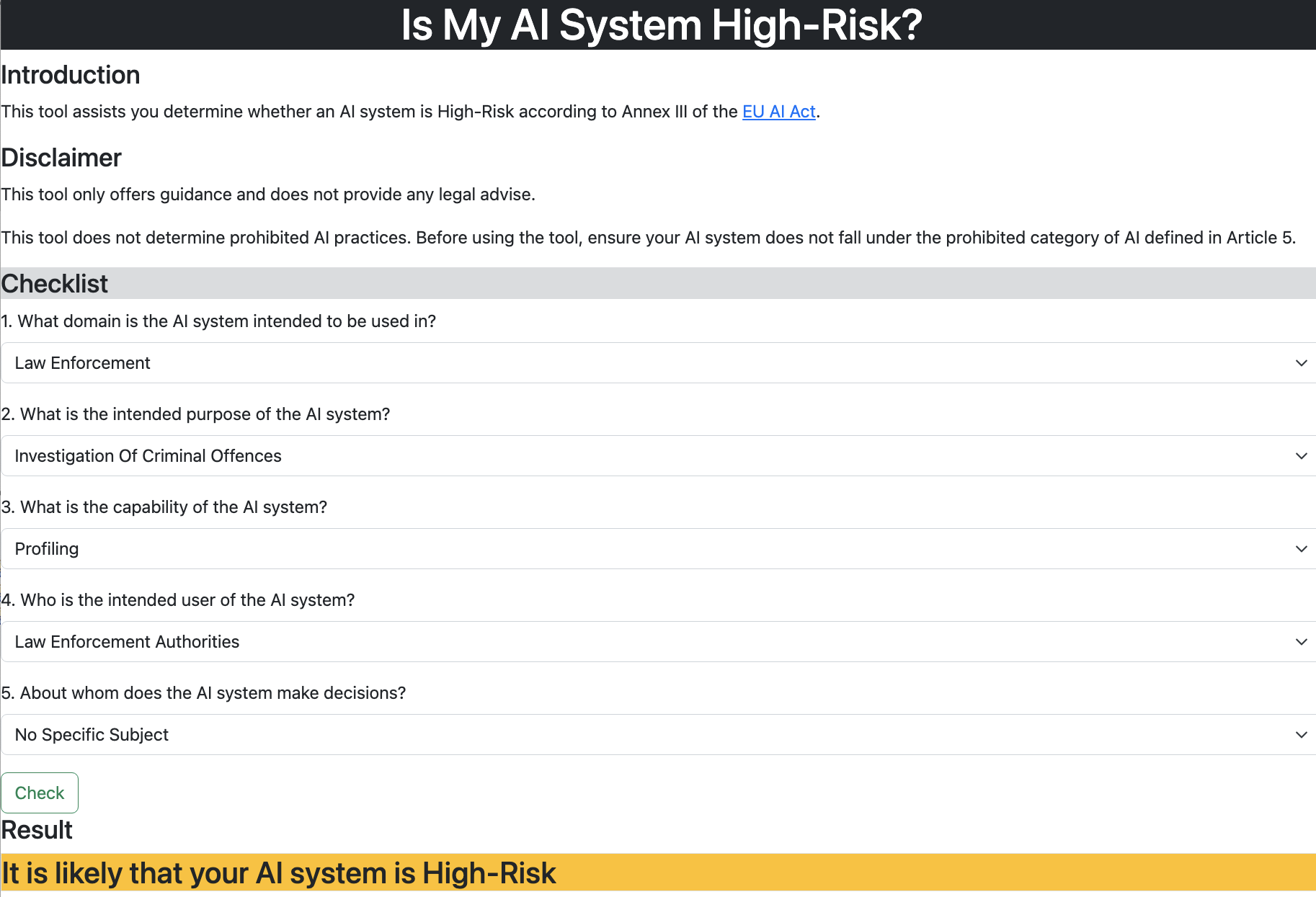
Substantial Modifications and Reviewing the High-Risk Assessment
Once classified as high-risk/not high-risk does not mean that the AI system will forever belong to the identified category. A key question for providers and users is when to revisit the decision regarding whether or not an AI system is high-risk. According to the AI Act, if an AI system undergoes “substantial modifications”, defined as changes that affect either the system’s conformity with the high-risk AI requirements or its intended purpose (Art. 3(23)), its life cycle will come to an end and the modified version is considered as a new system (Art. 3(1a)) and therefore requires a new assessment to determine if it is high-risk. An exception is made for substantial modifications in high-risk continuous learning systems (systems that continue to learn after being placed on the market or put into service) when the changes to the system and its performance are predicted, addressed, and documented in the initial conformity assessment (Art. 3(23)). It is not clear why foreseen substantial changes that are taken into account in conformity assessment of any high-risk AI system, regardless of its type, are not entitled to this exemption. Further, the line between modification and substantial modification is not clarified in the Act. Alternation of the intended purpose is explicitly indicated as substantial modification, yet it is not the only factor that affects the system’s conformity with the Act. Identification of cases of substantial modification is also needed for fulfilling record-keeping requirements as monitoring and recording of the factors that might result in substantial modifications in a high-risk AI system should be enabled through logging capabilities (Art. 12(2)(i)).
We recommend considering changes to the 5 concepts used for determining high-risk AI, namely domain, purpose, capability, user, and AI subject as substantial modifications due to their profound impacts on almost all of the requirements. However, this list is not exhaustive as there are other modifications that potentially affect conformity with the essential requirements, such as the examples listed in Table [tab:substantialmodification].
Modification of | Affected Requirement |
---|---|
Risk management process | Art. 9 Risk management system |
Training, validation, or testing data sets | Art. 10 Data and data governance |
Log management tools | Art. 12 Record-keeping |
Expected output | Art. 13 Transparency and provision of information to users |
Machine learning algorithms (that might lead to accuracy degradation) | Art. 15 Accuracy, robustness and cybersecurity |
Another trigger for re-assessment is the amendment of the high-risk areas, whose necessity is reviewed every 24 months after the regulation comes into force (Art. 84(1b)). The commission is granted the authority to add (Art. 7(1)) or remove (Art. 7(3)) high-risk applications listed in Annex III. It should be noted that only applications of AI listed under the 8 areas can be amended—denoting that the areas are not subject to amendments.
Responsible Body for Determination of High-Risk AI
Self-assessment of an AI system to determine whether it is high-risk, and in turn ensuring its compliance with the AI Act, are essentially the responsibility of the AI system provider (Art. 16(a))—an entity who “develops an AI system or that has an AI system developed and places that system on the market or puts it into service” (Art. 3(2)). However, under particular conditions (listed in Art. 23a(1)) this responsibility is delegated to other entities; for instance if an AI user, the entity “under whose authority the system is used.” (Art. 3(4)), modifies a non-high-risk AI system in such a way that after the modification it qualifies as high-risk, e.g. by alternating the intended purpose, then the user is subject to the providers’ obligations listed in Art. 16.
With the rise of general purpose AI systems, an important question is on whose shoulders the regulatory burdens should be. General purpose AI systems that may be used as a high-risk AI system or as its components should comply with high-risk AI requirements listed in Title III, Chapter 2 (Art. 4b(1)). According to Art. 4b(2), providers of such systems have to comply with some of the providers’ obligations, such as indicating their name and contact information (Art. 16(a)), ensuring the system undergoes conformity assessment procedures (Art. 16(e)), taking corrective actions when necessary (Art. 16(g)), affixing CE marking (Art. 16(i)), demonstrating conformity upon request (Art. 16(j)), drawing EU declaration of conformity (Art. 48), and establishing a post-market monitoring system (Art. 61). In addition, the general purpose AI providers should share necessary information required for compliance with the AI Act with “other providers intending to put into service or place such systems on the Union market as high-risk AI systems or as components of high-risk AI systems” (Art. 4b(5)), who are subject to obligations of high-risk AI providers according to Art. 23a(1)(e). However, if the provider of general purpose AI explicitly and genuinely excludes all high-risk uses, then the provider would be exempted from fulfilling the aforementioned requirements (Art. 4c). Considering the ongoing discussions in the European Parliament, stricter obligations are expected to be imposed upon general purpose AI systems and their providers in the final text of the AI Act.
Determining the subject of the AI Act’s legal requirements is also important in identification of parties potentially liable for the incidents caused by an AI system. According to the proposed AI Liability Directive [18], the high-risk AI provider, or any other entity who is subject to the providers’ obligations, as well as AI users would potentially be liable for damages caused by the high-risk AI due to its non-compliance with the AI Act’s requirements. Further research is required to address the abundance of question marks regarding liability, given the complexities in the AI value chain especially when general purpose AI is used.
VAIR: A Vocabulary of AI Risks
The high-level model of the 5 identified concepts is not sufficient for annotating AI use-cases, representing and documenting risk management, establishing rules for identification of high-risk AI, and sharing AI risk knowledge and best practices. These require enriching the model with instances of concepts represented formally and organised in hierarchies. State of the art regarding taxonomies for describing AI systems and their associated risks lacks structured representation of knowledge that can assist in discovering high-risk applications of AI, as shown in Table [tab:high-risk criteria in taxonomies].
With multiple and changing high-risk classifications and the unknown land of AI risks which yet has to be explored, there is a need for an open, extensible, and machine-readable vocabulary. In this section, we represent the vocabulary of AI Risks (VAIR)—a formal taxonomy to represent hierarchies of AI and risk concepts.
Taxonomy | 1. domain | 2. purpose | 3. capability | 4. user | 5. AI subject |
---|---|---|---|---|---|
AIAAIC | Sector | Purpose | — | — | — |
AIID | Sector of deployment | — | AI functions and applications | — | — |
AITopics | Industry | — | — | — | — |
OECD taxonomy | Industrial sector | — | AI system task | User | Impacted stakeholder |
AIRO | Domain | Purpose | AI capability | AI user | AI subject |
Overview of VAIR
VAIR provides semantic specifications for cataloguing AI risks in a FAIR (Findable, Accessible, Interoperable, Reusable) manner. It reuses core concepts of AIRO [11] as its foundation and represents instances using the SKOS (Simple Knowledge Organization System) model7. In creation of VAIR, we considered rules suggested by Poveda-Villalón et al. [19] to ensure its FAIRness. VAIR is published online as an open resource under the CC-By-4.0 licence at https://w3id.org/vair. In the current iteration of development, the AI Act, ISO/IEC 22989:2022 on AI terminology8, and the AI Watch’s AI taxonomy [20] were used as primary resources for identification and interpretation of concepts. For the sake of simplicity, VAIR incorporates the following modules:
AI: contains taxonomies of techniques (number of instances in the taxonomy: 19), capabilities (30), types of AI (17), components (34), life cycle phases (13), characteristics (20) including trustworthiness characteristics, and outputs (6).
Use of AI: includes taxonomies for defining AI use-cases namely purposes (114) and domains (13).
Risk: contains risk sources (43), consequences (4), impacts (12), controls (18), and impacted areas (5) taxonomies.
Stakeholder: contains stakeholder roles (40) with a focus on taxonomies for AI subjects and AI users.
Document and standard: contains a list of technical documents (12) including those required for conformity assessments and standards (22) that can be used in implementation of the AI Act.
VAIR Applications and Benefits
VAIR contains the concepts required for specifying Annex III conditions (represented in Figure [fig:conditions]) and therefore can be used for creating rules for determining high-risk AI and checking them for partial to full applicability to AI use-cases in a logical and automated manner. Using the vocabulary, detailed modelling of AI systems and of the information related to AI risk management, and generating machine-readable documentation would be possible. VAIR enables easy and free access to information regarding AI risks, impacts, and mitigation measures, and therefore can be served as a helpful resource in performing AI risk management and impact assessment tasks. Using VAIR alongside existing vocabularies that concern risk, such as the Data Privacy Vocabulary (DPV)9, facilitates integration of existing risk management and impact assessment practices when dealing with multiple EU regulations and conducting shared impact assessments [21]. VAIR supports interoperability in the AI ecosystem by providing a standardised and formal way of describing AI risks. In addition, reuse and enhancement of the vocabulary over time by different stakeholders to include the risks that emerge over time and further extension of the vocabulary to create domain-specific taxonomies of AI risks would be possible. Organising information through class hierarchies enables specification of generic and more specific risks which helps in drawing the boundaries between general and domain-specific risks. This is helpful in addressing the liability pressure faced by providers for using general purpose AI by enabling the users to distinguish risks caused by use of a general AI system and risks associated with the context or purpose of the application.
VAIR Limitations and Plans for Enhancement
VAIR is an ongoing effort to provide a reference AI risk taxonomy. The current iteration of VAIR reflects concepts from the AI Act, ISO/IEC 22989, and AI Watch’s taxonomy. The reviewed taxonomies in Section 2.3 are useful resources for extending VAIR, however, reusing them for population of the vocabulary requires further work to ensure the definition of their high-level concepts are consistent with the definitions in the vocabulary resolving any conflicts or inconsistencies that may arise from integrating the taxonomies.
This version only includes sub-class relationship between concepts, providing related relations which can assist in identification of AI risk-related patterns such as technique-risk, domain-impacted stakeholder, and risk-mitigation is considered as future work. These patterns can form a primary checklist for AI risk management as a starting point for risk identification and mitigation. Different stakeholders have not been involved in creation of the vocabulary yet. Before this involvement, mechanisms for conflict resolution and governance as well as arrangements for extending the vocabulary should be established.
Harmonised Standards and Conformity with the AI Act’s Obligations
The AI Act specifies the conditions for high-risk AI systems (Art. 6), prescribes the requirements for those systems (Title III, Chapter 2), and defines obligations for their providers (Title III, Chapter 3); but it does not indicate how the regulation should be implemented in practice, this is to ensure the Act’s flexibility and avoid over-regulation. However, to help high-risk AI providers, the Act suggests using harmonised standards as means for alleviating conformity tasks. Although compliance with these standards is not enforced [22], when a high-risk AI conforms to the harmonised standards, indexed in the Official Journal of the European Union, its conformity with the Title III, Chapter 2 requirements is presumed (Art. 40(1)). In the draft standardisation request [23], the Commission has called upon CEN (European Committee for Standardisation) and CENELEC (European Committee for Electrotechnical Standardisation) to develop required harmonised standards. With a deadline in early 2025, CEN and CENELEC are delegated to create European standard(s) and/or European standardisation deliverable(s) in 10 areas, including AI risk management systems.
Within this area, ISO/IEC 23894 “Artificial intelligence — Guidance on risk management”10, published in February 2023, is a dominant standard that aims to guide organisations in managing AI risks through integration of risk management tasks into AI development tasks or any activity that incorporate AI. Table [tab:riskmanagement] shows the alignment of the AI Act’s risk management system steps (Art. 9(2)) with ISO/IEC 23894’s risk management process.
Given that this standard is an extension of the ISO’s generic risk management standard (ISO 3100:201811), it is inherently non-prescriptive, therefore could not be used as a reference for AI risk management system certification. Additionally, it focuses on organisational risk [24], whilst fulfilment of the risk management requirements, referred to in Art. 9, requires addressing risks to external stakeholders’ health, safety, and fundamental rights. To address this concern, a new work item is proposed in CEN-CENELEC to create a checklist for AI risks management (CLAIRM), whose core is a non-exhaustive list of AI risks, risk sources, impacts, and suitable mitigation measures. In addition to a checklist of risk criteria, providing concrete guidelines as well as best practices is under consideration. Although CLAIRM might resolve the issue with the scope, the concern regarding certifiability remains valid.
AI Act Art. 9 clause | ISO/IEC 23894 |
---|---|
(2a) identification and analysis of the known and foreseeable risks | 6.4.2 Risk identification .3 Risk analysis |
(2c) evaluation of other possibly arising risks | 6.4.4 Risk evaluation Monitoring and review |
(2d) adoption of suitable risk management measures | 6.5 Risk treatment |
(1) AI risk management documentation | 6.7 Recording and reporting |
As AI risks are context-dependent, In addition to horizontal standards, vertical specifications, which lay down domain-specific guidelines, principles, and norms, are required to support providers of AI systems in different domains, in particular the Annex III areas. Relevant to biometrics (Annex III, pt. 1), ISO/IEC CD 9868 “Remote biometric identification systems — Design, development, and audit”12, wherein many of the AI Act’s requirements including risk management will be addressed, is in early stages of development. This future standard will touch upon technical solutions, development practices, and post-development monitoring and auditing.
Adequacy of European Standards for Compliance with High-Risk AI Requirements
It is evident that presently there are not sufficient European standards to fulfil the Commission’s request. Since the publication of the AI Act’s proposal, the Joint Research Centre (JRC), the European Commission’s science and knowledge service, has provided two comprehensive analyses of the AI standardisation landscape to examine sufficiency and suitability of published and under-development AI standards for conformity to the Act’s requirements. In the first report [25], published in 2021, a high-level mapping of relevant standards, developed by international and European standardisation bodies, namely ISO/IEC, CEN-CENELEC, ITU-T, ETSI, and IEEE, to high-risk AI requirements is presented. To identify the most relevant standards to each requirement a metric, called suitability index (Si) is used to quantify adequacy of standards for supporting the Act’s requirements based on the following criteria: domain generality, compliance management, typology, and maturity. In the second report [24], published in 2023, the focus is on the alignment of the Act’s high-risk obligations with two families of IEEE Standards: 7000 series on ethical concerns and the Ethics Certification Program for Autonomous and Intelligent Systems (ECPAIS). Assessing the extent of alignment is carried out based on the four criteria mentioned above in addition to the criteria listed below: AI coverage, maturity and technical detail, gaps and complementarities, and relevant standards.
Overview of the Current State of Standardisation in ISO/IEC JTC 1/SC 42
To reflect the gap between the current state of AI standardisation at an international level and the desired state of EU harmonised standards required for compliance with the AI Act, we map standardisation activities undertaken by ISO/IEC JTC 1/SC 42 into the high-risk AI requirements. Table [tab:standards] lists JTC 1/ SC 42 published and under-development standards, their development stage, type, and coverage, alongside the AI Act’s requirements they address. It should be noted that the table excludes foundational AI standards including ISO/IEC 22989:2022 AI concepts and terminology, ISO/IEC 23053:2022 framework for ML-based AI systems, and ISO/IEC TR 24372:2021 overview of computational approaches for AI. Our analysis demonstrates the following challenges: (i) there is a lack of standards to address requirements regarding creation of documents, such as technical documentation (Art. 11) and instructions for use (Art. 13), as well as record-keeping (Art. 12), (ii) there is a paucity of organisational and certifiable standards as the only certifiable standard on the list is ISO/IEC 42001 on AI management systems. Therefore, a key issue with the future harmonised AI standards is how to benefit from the presumption of conformity and demonstrate conformance to non-certifiable standards, (iii) currently all of the reviewed ISO standards are behind paywalls and gaining access to harmonised standards would be a critical problem, especially for startups, SMEs, and research institutions.
Area | AI Act | Standard (ISO development stage as of April 2023) | Type | Coverage |
---|---|---|---|---|
Determine high-risk AI | Art. 6 | ISO/IEC TR 24030:2021 AI — Use cases (90.92) | Guidance | AI uses |
ISO/IEC DIS 5339 Guidance for AI applications (40.20) | Guidance | AI uses | ||
Risk management system for AI systems | Art. 9 | ISO/IEC 23894 Guidance on risk management | Guidance | AI system |
ISO/IEC TR 24027:2021 Bias in AI systems and AI aided decision making | Technical | AI system | ||
ISO/IEC TR 24368:2022 Overview of ethical and societal concerns | Guidance | AI system | ||
ISO/IEC AWI 42005 AI system impact assessment (20.0) | Guidance | AI system | ||
ISO/IEC CD TR 5469 Functional safety and AI systems (30.60) | Guidance | AI system | ||
ISO/IEC CD TS 12791 Treatment of unwanted bias in classification and regression ML tasks (30.20) | Technical | Machine learning | ||
Data governance and quality | Art. 10 | ISO/IEC 20546:2019 Big data — Overview and vocabulary | Foundational | Big data |
ISO/IEC TR 20547 series Big data reference architecture | Technical | Big data | ||
ISO/IEC 24668:2022 Process management framework for big data analytics | Organisational | Big data | ||
ISO/IEC FDIS 8183 Data life cycle framework (50.20) | Guidance | Data | ||
ISO/IEC [CD/DIS] 5259 series Data quality for analytics and ML (different stages) | Technical | Data | ||
Transparency | Art.13 | ISO/IEC AWI 12792 Transparency taxonomy of AI systems (20.00) | Guidance | AI systems |
ISO/IEC AWI TS 6254 Objectives and approaches for explainability of ML models and AI systems (20.00) | Guidance | AI systems ML models | ||
Human oversight | Art. 14 | ISO/IEC WD TS 8200 Controllability of automated AI systems (20.60) | Technical | AI system |
System quality | Art. 15 | ISO/IEC TR 24028:2020 Overview of trustworthiness in AI | Technical | AI system |
ISO/IEC WD TS 25058 SQuaRE — Guidance for quality evaluation of AI systems (20.60) | Technical | AI system | ||
ISO/IEC PRF TS 25059 SQuaRE — Quality model for AI systems (50.20) | Technical | AI system | ||
ISO/IEC AWI TS 29119-11 Testing of AI systems (20.00) | Technical | AI system | ||
ISO/IEC TS 4213:2022 Assessment of machine learning classification performance | Technical | Machine learning | ||
ISO/IEC TR 24029 Assessment of the robustness of neural networks | Technical | Neural networks | ||
ISO/IEC AWI TS 17847 Verification and validation analysis of AI (20.00) | Technical | AI system | ||
Quality management system | Art. 17 | ISO/IEC DIS 42001 Management system (40.60) | Organisational | Management system |
Conclusion
Within the EU AI Act’s multi-layered risk-based approach, high-risk AI is the key category on which the majority of obligations are incurred. In this paper, we provide a simplified framework for discovery of high-risk AI use-cases, referred to in Annex III, by identifying 5 core concepts namely: domain, purpose, AI capability, AI user, and AI subject. We argued that these concepts can also be considered as the main factors whose alternation would result in substantial modifications. To enable automation and integration in AI risk management tasks and promote knowledge sharing and interoperability between AI stakeholders, we presented VAIR as a formal taxonomy for AI risk-related concepts. With further ongoing enhancements, VAIR would serve as a checklist for AI risk identification, evaluation, and management. Given the key role of harmonised standards in implementation of the AI Act, we analysed the implications of the Act’s use of standards and the adequacy of ISO/IEC JTC1/SC42 AI standards in addressing high-risk requirements.
Funding: This project has received funding from the European Union’s Horizon 2020 research and innovation programme under the Marie Skłodowska-Curie grant agreement No 813497 (PROTECT ITN), as part of the ADAPT SFI Centre for Digital Media Technology is funded by Science Foundation Ireland through the SFI Research Centres Programme and is co-funded under the European Regional Development Fund (ERDF) through Grant#13/RC/2106_P2.
Thanks: We thank Víctor Rodríguez-Doncel for his support in development of the tool.
References
https://www.europarl.europa.eu/olp/en/ordinary-legislative-procedure/overview↩︎
See the Commission’s proposal here: https://eur-lex.europa.eu/legal-content/EN/TXT/?uri=celex%3A52021PC0206↩︎
See the French presidency version here: https://artificialintelligenceact.eu/wp-content/uploads/2022/06/AIA-FRA-Consolidated-Version-15-June.pdf↩︎