Investigating Conditional Data Value Under GDPR
Workshop on Governing Value: The Practice of Exploiting Data Value (DataValue) - co-located with International Conference on Semantic Systems (SEMANTiCS)
✍ Harshvardhan J. Pandit* , Declan O'Sullivan , Dave Lewis
Description: Discussing the impact of GDPR's consent on the value of data and how this can be used to better utilise available data
published version 🔓open-access archives: harshp.com , TARA , zenodo
📦resources: Investigating Conditional Data Value Under GDPR
Abstract The calculation of data value is based on the assumption of continued presence of information. When the data in consideration concerns personal information, laws such as the European General Data Protection Regulation (GDPR) affect how this data can be exploited. Under the GDPR, data must be used on the basis of explicit consent, which can be withdrawn at any time by the concerned data subject. This posits that personal data dependant on consent can not be used after the consent is withdrawn. The data value associated with such personal data will also change with such a change in consent. This position paper explores the change in data value for personal data under the GDPR. The paper analyses the obligations and conditions provided by the GDPR for data usage and how they affect the data value chain. This is then used to identify potential approaches towards effective utilisation of available data through techniques such as aggregation and anonymisation. Finally, the paper proposes a method for investigating conditional data value by incorporating data existence and availability as a metric.
Introduction
Personal data is a valuable asset for organisations at all scales and globally. The monetisation of personal data is possible using various approaches, from being used to provide free online services in exchange for private information [1], to being used as an artefact with quantifiable monetary value [2], [3]. This value of personal data combined with the feasibility of big data provides a mechanism for its exploitation at scale. This model is seen to be successful from the rise of internet giants such as Facebook and Google which both provide advertisements based on personal data as their primary revenue stream. A study by JPMorgan Chase in 2012 found that each unique user is worth roughly $4 to Facebook and $24 to Google [4]. This provides a monetary and quantifiable motivation for such organisations to collect and retain as much personal data as possible. Acxiom, the largest data broker, targets this niche by collecting personal data from about 700 million users worldwide and selling aggregate statistics to top companies [5]. Such instances highlight the intricate relationship between the revenue of a company with the value of data it holds. It also serves to highlight that pricing personal data is about datasets and not personal data itself [3].
When calculating the value of such data, the assumption of availability is constant - that is, the data is assumed to forever be available within the data value chain. However, when this data contains personal information, or other types of sensitive information, its availability is dependant on territorial laws that can restrict its collection and usage.
The General Data Protection Regulation (GDPR), an European law, is applicable to any organisation that uses data containing personal information, and stipulates several conditions and obligations that must be fulfilled towards its compliance. One of its important obligations is the mechanism of consent given by the data subject which is required for all personal data and activities deemed non-essential to the provided services. Additionally, consent given in the past can be withdrawn at any time in the future as per the rights provided by GDPR. Activities that require such given consent as their legal justification can no longer utilise the personal data after the consent has been withdrawn. Also, any addition or change in such activities may necessitate a renewal of consent from the data subject, which prevents personal data from being misused under false pretexts. Compliance towards these obligations is an important matter for organisations due to the significant fines of 4% of global turnover or 20 million euros, whichever is higher.
Currently, the industry is undergoing a paradigm shift over the use of active choice models where users pay for a service through a monetary value or with their personal data, which is incompatible with the requirements of the GDPR [3], [6]. In addition to this, GDPR provides rights for data subject to change their given consent which can change or restrict the operations on their personal data at any time. Existing methods for expressing data value [7]–[11], including using a data value chain [12] or network [13] or meta-model [14], do not explicitly take into account this conditional availability of data. This is due to accessibility (which implies availability) being a core dimension of data quality, [15], and therefore data value.
From the data value perspective, personal data under the GDPR can only be exploited to the extent permitted by its associated given consent. Furthermore, the availability of this data is also dependant on the continuation of the given consent, where the data subject has the power to withdraw it any time. Through this position paper, we aim to highlight this conditionality of personal data and its effects on inclusion and exploitation in a data value chain. We start by discussing the impact of GDPR on the value of personal data as an asset in Section 2, We present our ideas towards an approach based on data availability in Section 3, and conclude the paper in Section 4 with possible avenues for future work.
Impact of GDPR
While the GDPR has a significant number of obligations, we only focus on those that affect the availability of personal data. Primary amongst these is the given consent (Article 7), with others being the Right to be forgotten (Article 17) and Right to data portability (Article 20). We present here our analysis of their effects on the data value of personal data.
Given Consent
The given consent defines what activities can be performed on the personal data it is associated with. Since this consent is to be acquired before the actual collection of personal data, it can limit the data that is available for a specific purpose. For example, if the data subject refuses to grant consent to use their personal data for advertising activities, the data cannot be used for advertising even though it is collected and used for other purposes.The right to withdraw consent, as provided by the GDPR, allows the data subject to change or withdraw their previously given consent at any time. Therefore, data already collected and being used based on the given consent for some purpose may subsequently become unavailable due to the withdrawal of this consent. In the earlier example, if the data subject had consented to the use of their data for advertising purposes, then the data would only be available for use in advertising activities as long as the data subject does not withdraw this consent.
The above represent two different events or points in time based on consent that affect the availability of data. The first represents a conditionality based on usage where the value cannot be incorporated as the data cannot be used. An example of this for the advertising use-case is where anonymisation or aggregate statistics are not be possible due to the data not being collected. The second conditionality arises when data is already being used but can no longer be used due to the withdrawal of consent. In this case, the value of data is present and can be exploited until the consent is withdrawn. During this time, it is possible (under the GDPR) to generate anonymised datasets and aggregate statistics as long as they are not deemed to contain personally identifiable information.
Right to be Forgotten
GDPR allows data subjects the right to have their personal data erased based on a number of conditions (not listed in this paper). The right to be forgotten is closely related to other obligations of the GDPR, such as the right to withdraw consent, and therefore complements many of its processes. For example, the data subject can request their data to be erased after withdrawing their consent. In this case, from the data value perspective for the advertising activities, the (un-)availability remains the same. In both cases, changes to the availability of data may happen at any time upon exercising of rights.
Anonymisation & Pseudo-Anonymisation
If the personal data is anonymised, and stripped of all personally identifiable information, then it can be used without being bound to consent. This data (if it is no longer personally identifiable) can be used in the data value chain without conditionality. However, anonymisation here refers to complete anonymisation, which is difficult to achieve in practice. Pseudo-anonymisation, where personal data is partially anonymised, can be a technically as well as legally feasible solution to this problem [16]. Under the GDPR, pseudo-anonymised data which cannot be de-anonymised by the organisation without additional external information can be effectively treated as anonymised data only for activities within the organisation. The value for such data is dependant on whether the data can be de-anonymised and if it can be retained after the removal of the personal data it was generated from. In the latter case, the pseudo-anonymised data can continue to be utilised in the data value chain.
Obligations for Controllers & Processors
Under the GDPR, any exercise of rights or changes in consent must be propagated from the Data Controller to any other Controllers or Processors that are acting on the affected data. Consider the scenario where a Data Controller provides the Data Processor with personal data to perform analytics and aggregate statistics for advertising. If the Data Subject exercises their right which prohibits these activities, the Data Controller can no longer provide this data to the Data Processor. This affects the value of the data returned by the Data Processor. Therefore, under the GDPR, effects propagate along a chain of entities based on their role (Controller or Processor) and can affect the data value of services they provide.
Incorporating Conditionality in Data Value
Temporal changes to personal data are an issue regarding its consistent exploitation. Under the GDPR, the availability of personal data itself becomes an issue which is not currently associated with data value. We propose to incorporate this conditionality into data value characterisations and representations. Our preferred approach for this is to model data availability as a metric to determine its value. Availability as a metric works on two scenarios: first, where the data may not be available from the start; second, where the data may become unavailable in the future. Whether these two should be separate metrics or can be represented through a single concept is an investigation we intend to carry out as part of the future work.
Our initial efforts investigate incorporating data availability into existing methods for modelling data value. One such approach is to use the data-based value framework [9] which models data-based value creation into the four areas of (i) data collection, (ii) information creation, (iii) value creation, and (iv) distribution through the provider network. These are further divided into nine factors based on analysis and design of information-intensive services. With the inclusion of availability as an additional factor, we have the ten factors for data-based value creation: (1) data source, (2) data collection, (3) data (artefact), (4) data analysis, (5) information on the data source, (6) information delivery, (7) customer (information user), (8) value in information use, (9) provider network, and (10) data availability.
Incorporating conditionality through the availability metric into the framework for data-based value creation allows modelling of information with its consistency in terms of availability. It also allows modelling of events that may affect data value such as withdrawal of consent under GDPR. This argument is not applicable for cases such as those based on open data [13], [17] which incorporate explicit availability in their methodology.
In terms of assessment of data value, a practical method for using conditionality is using a selective approach based on availability. This is relevant for cases where the data can be aggregated or (pseudo-)anonymised when available. In a data value chain, the value generated from only the aggregated or pseudo-anonymised data is always a subset of the total value generated when the complete data is available. By identifying which data is conditionally available along with the specific events associated with this conditionality, it is possible to target approaches that are better for exploiting data value. As an example, consider the case where the data subject can withdraw their consent for a specific process such as advertising. When modelling the data value for this process, the data may either be unavailable (consent not given), available (consent given), or may become unavailable (consent withdrawn). Of these three events, only the latter two allow data value to be generated based on availability of data. Of these, when data is available (with given consent), its value can be represented as a continuous function along time. When consent is withdrawn and the data becomes unavailable, its value based on availability drops to zero and becomes non-existent.
An analysis of such events and their effects is the first step towards identifying alternate means of exploiting data value. For the earlier example, aggregation and (pseudo-)anonymisation are mechanisms that can sustain the data value beyond its availability. To maximise their usage, these processes need to be re-analysed in terms of their dependency on data with respect to its availability. By explicitly incorporating the unavailability of data, approaches can better exploit data through secondary functions such as aggregation and anonymisation that allow some data value to persist beyond its availability.
The modelling of data value in terms of availability also serves to identify services and processes that may be affected by its conditionality. Data that is conditionally available has a transitive conditional value that propagates to processes that depend on usage of such data. Under this viewpoint, processes that use conditionally available data will provide conditional value. To identify such processes and maximise approaches for data value generation, we propose to use a pruning approach for removing non-effective methods. Consider a tree-based representation, as depicted in Fig. 1, of all possible events and its effects on the availability of data. In this representation, the root of the tree is the data instance in question, and is connected to the services that depend on it through the various events that affect its availability. By pruning branches where data may not be available (coloured green, with dashed lines) or may become unavailable (coloured red, with thicker lines), it is possible to identify specific areas of focus to maximise value. We intend to formalise this method as part of our planned future work of representing conditional data value.
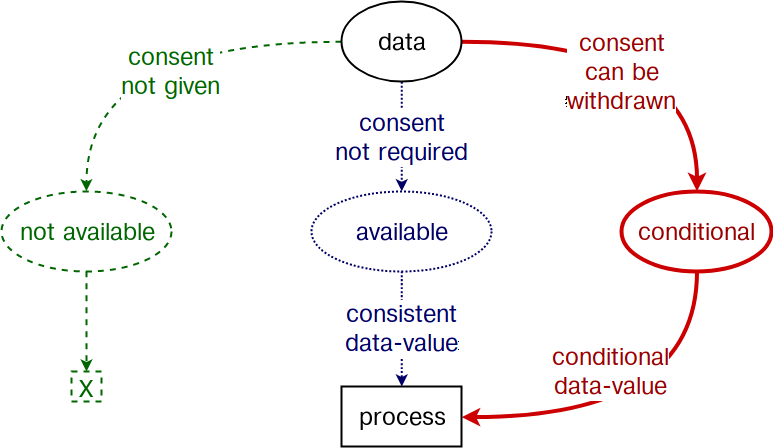
Conclusion
Through this position paper, we have highlighted the effect of the General Data Protection Regulation (GDPR) on the availability of personal data and its subsequent impact on data value. The analysis of GDPR in this paper focuses on the facility of the given consent and various rights to restrict the usage and availability of personal data. This is used to highlight the conditionality of data and its effects on availability to processes that use it in a data value chain. The contribution of the paper is the proposed approach for using availability as a metric which allows modelling conditional data value. A discussion of incorporating this metric into an existing framework for data-based value [9] is provided. The use of availability to highlight alternate means of data value exploitation for personal data such as aggregation and anonymisation are also explored.
In terms of future work, the paper highlights the need to formalise the presented approach towards representing conditionality of data by using availability as a metric. A tree based pruning method for identifying processes that can be better exploited in terms of data availability is also discussed. The role of methods such as aggregation and anonymisation in exploiting data when it may not be available is crucial in terms of exploiting data value and needs to be incorporated into data value chains that use personal data. This can be extended to other relations relevant to the use and sharing of personal data, such as between Controllers and Processors, which also need to incorporate the relationship between data value and availability of data.
Acknowledgements
This work is supported by the ADAPT Centre for Digital Content Technology which is funded under the SFI Research Centres Programme (Grant 13/RC/2106) and is co-funded under the European Regional Development Fund.
References
[1] C. Niu, Z. Zheng, F. Wu, S. Tang, X. Gao, and G. Chen, “Unlocking the Value of Privacy: Trading Aggregate Statistics over Private Correlated Data,” in Proceedings of the 24th ACM SIGKDD International Conference on Knowledge Discovery & Data Mining, 2018, pp. 2031–2040, doi: 10.1145/3219819.3220013 [Online]. Available: http://doi.acm.org/10.1145/3219819.3220013. [Accessed: 24-Jul-2018]
[2] C. Li, D. Y. Li, G. Miklau, and D. Suciu, “A Theory of Pricing Private Data,” Commun. ACM, vol. 60, no. 12, pp. 79–86, Nov. 2017, doi: 10/gdvm3k. [Online]. Available: http://doi.acm.org/10.1145/3139457. [Accessed: 24-Jul-2018]
[3] G. Malgieri and B. Custers, “Pricing privacy – the right to know the value of your personal data,” Computer Law & Security Review, vol. 34, no. 2, pp. 289–303, Apr. 2018, doi: 10/gc7nbt. [Online]. Available: http://linkinghub.elsevier.com/retrieve/pii/S0267364917302819. [Accessed: 19-Jul-2018]
[4] J. Brustein, “Start-Ups Aim to Help Users Put a Price on Their Personal Data,” The New York Times, Feb. 2012 [Online]. Available: https://www.nytimes.com/2012/02/13/technology/start-ups-aim-to-help-users-put-a-price-on-their-personal-data.html. [Accessed: 24-Jul-2018]
[6] P. Hacker and B. Petkova, “Reining in the Big Promise of Big Data: Transparency, Inequality, and New Regulatory Frontiers,” Social Science Research Network, Rochester, NY, SSRN Scholarly Paper ID 2773527, May 2016 [Online]. Available: https://papers.ssrn.com/abstract=2773527. [Accessed: 25-Jul-2018]
[7] J. Attard and R. Brennan, “A Semantic Data Value Vocabulary Supporting Data Value Assessment and Measurement Integration,” in Proceedings of the 20th International Conference on Enterprise Information Systems, ICEIS 2018, Funchal, Madeira, Portugal, March 21-24, 2018, Volume 2, 2018, pp. 133–144, doi: 10.5220/0006777701330144 [Online]. Available: http://www.scitepress.org/DigitalLibrary/Link.aspx?doi=10.5220%2f0006777701330144. [Accessed: 07-Jul-2018]
[8] L. Kugler, “The War over the Value of Personal Data,” Commun. ACM, vol. 61, no. 2, pp. 17–19, Jan. 2018, doi: 10/gdsgzm. [Online]. Available: http://doi.acm.org/10.1145/3171580. [Accessed: 24-Jul-2018]
[9] C. Lim, K.-H. Kim, M.-J. Kim, J.-Y. Heo, K.-J. Kim, and P. P. Maglio, “From data to value: A nine-factor framework for data-based value creation in information-intensive services,” International Journal of Information Management, vol. 39, pp. 121–135, Apr. 2018, doi: 10/gc9krt. [Online]. Available: http://www.sciencedirect.com/science/article/pii/S0268401217300816. [Accessed: 24-Jul-2018]
[10] S. Spiekermann and J. Korunovska, “Towards a value theory for personal data,” Journal of Information Technology, vol. 32, no. 1, pp. 62–84, Mar. 2017, doi: 10/gdvm22. [Online]. Available: https://link.springer.com/article/10.1057/jit.2016.4. [Accessed: 24-Jul-2018]
[11] N. Tempini, “Till data do us part: Understanding data-based value creation in data-intensive infrastructures,” Information and Organization, vol. 27, no. 4, pp. 191–210, Dec. 2017, doi: 10/gcr2xb. [Online]. Available: http://www.sciencedirect.com/science/article/pii/S1471772716300653. [Accessed: 24-Jul-2018]
[12] A. Latif, A. U. Saeed, P. Hoefler, A. Stocker, and C. Wagner, “The Linked Data Value Chain: A Lightweight Model for Business Engineers.” in I-SEMANTICS, 2009, pp. 568–575.
[13] J. Attard, F. Orlandi, and S. Auer, “Exploiting the Value of Data through Data Value Networks,” 2017, pp. 475–484, doi: 10.1145/3047273.3047299 [Online]. Available: http://dl.acm.org/citation.cfm?doid=3047273.3047299. [Accessed: 07-Jul-2018]
[14] M. I. S. Oliveira, L. E. R. A. Oliveira, M. G. R. Batista, and B. F. Lóscio, “Towards a meta-model for data ecosystems,” 2018, pp. 1–10, doi: 10.1145/3209281.3209333 [Online]. Available: http://dl.acm.org/citation.cfm?doid=3209281.3209333. [Accessed: 07-Jul-2018]
[15] D. Laney, “Infonomics: The economics of information and principles of information asset management,” in The fifth mit information quality industry symposium. Cambridge, 2011, vol. 9, p. 2.
[16] M. Hintze and G. LaFever, “Meeting Upcoming GDPR Requirements While Maximizing the Full Value of Data Analytics,” 2017, doi: 10/gdtm46. [Online]. Available: https://papers.ssrn.com/sol3/papers.cfm?abstract_id=2927540. [Accessed: 24-May-2017]
[17] J. Attard, F. Orlandi, and S. Auer, “Value Creation on Open Government Data,” in 2016 49th Hawaii International Conference on System Sciences (HICSS), 2016, pp. 2605–2614, doi: 10.1109/HICSS.2016.326.