An Ontology for Standardising Trustworthy AI
Factoring Ethics in Technology, Policy Making and Regulation
✍ Dave Lewis* , David Filip , Harshvardhan J. Pandit
Description: Presents a simple ontology that can be used for checking the consistency and overlap of concepts from different standards, regulations and policies for Trustworthy AI.
published version 🔓open-access archives: harshp.com , TARA , zenodo
Abstract
Worldwide, there are a multiplicity of parallel activities being undertaken in developing international standards, regulations and individual organisational policies related to AI and its trustworthiness characteristics. The current lack of mappings between these activities presents the danger of a highly fragmented global landscape emerging in AI trustworthiness. This could present society, government and industry with competing standards, regulations and organisational practices that will then serve to undermine rather than build trust in AI. This chapter presents a simple ontology that can be used for checking the consistency and overlap of concepts from different standards, regulations and policies. The concepts in this ontology are grounded in an overview of AI standardisation currently being undertaken in ISO/IEC JTC 1/SC 42 and identifies its project to define an AI management system standard (AIMS or ISO/IEC WD 42001) as the starting point for establishing conceptual mapping between different initiatives. We propose a minimal, high level ontology for the support of conceptual mapping between different documents and show in the first instance how this can help map out the overlaps and gaps between and among SC 42 standards currently under development.
Keywords: Trustworthy AI, AI Ethics, AI Governance, AI Standards, Ontology
Introduction
To be effective, future regulation and organisational policy aimed at achieving trustworthy AI must be supported by some degree of standardisation in processes and technological interoperability. The rapid development of AI technologies and the growth of investment in AI applications presents a pacing problem, wherein the rapid change in characteristics of AI related to policy and regulatory issues outpaces ability of societies to legislate for or regulate the technology. At the same time, the multinational nature of the major commercial developers of AI, plus the expanding access to AI skills and computing resources means that standards must be agreed internationally to be of widespread use in supporting policy and regulations. While there has been an explosion in policy documents from national authorities, international organisations and the private sector on the ethical implications of AI, standards in this area have been slower to emerge. Understanding existing standardised ICT development and organizational management practices offer insight into the extent to which they may provide a basis for standardising practice in governing the development and use of more trustworthy and ethical AI. Standards Developing Organizations (SDOs) vary in their approach to addressing specific ethical issues.
The Institute of Electrical and Electronic Engineers (IEEE) global initiative on ethically aligned design for autonomous and intelligent systems has spawned the IEEE 7000 standards working group that places ethical issues at its heart [1]. This work was seeded from a set of principles defined in a comprehensive international export review on Ethically Aligned Design [2], which also highlighted the influence of classical ethics, professional ethics and different moral worldviews
A different approach is taken by the ISO/IEC Joint Technical Committee 1 (JTC1). JTC1 which was established by the International Standardization Organization (ISO) and the International Electro-technical Commission (IEC) in 1987 to develop, maintain and promote standards in the fields of Information Technology (IT) and Information and Communications Technology (ICT). Expert contributions are made via national standards bodies and documents (over 3000 to date) are often used as technical interoperability and process guideline standards in national policies and international treaties, as well as being widely adopted by companies worldwide. Statements of relevance to UN Sustainable Development Goals and Social Responsibility Guidelines are an inherent part of all new standardisation projects proposed in JTC 1 [3]. AI standards are addressed together with big data technology standards by the JTC 1 subcommittee (SC) 42 which was first chartered in autumn 2017 and held its inaugural meeting in April 2018. As of the end of 2020 it has published six standards and has active projects addressing 23 others[1].
This chapter highlights the challenges facing companies and authorities worldwide in advancing from the growing body of work on ethical and trustworthy AI principles to a consensus on organisational practices that can deliver on these principles across the global marketplace for AI-based ICT. We review how SC 42 standardisation efforts benefit from building on established process standards in areas of management systems, IT governance, risk and system engineering. From this analysis, we identify a simple conceptual model that can be used to capture the semantic mapping between different SC 42 standards. An ontology is used as it allows a conceptual model to be defined that links together concepts via association into a network of concepts. This has the potential to establish an open ontology that can map between core concepts from standardization and pre-standardization deliverables in varied states development, formal approval, and international community consensus with concepts needed to address trustworthy AI. Such a network allows the definition of terms and concepts from different standards related documents to be interlinked and thereby the consistency of conceptual use between different can be analysed and improvements suggested. While this is not intended to replace the consistency checking that occurs naturally in the JTC1 standards development process, it does allow us to identify some mapping and comparisons between different forms of standard that have been applied to different areas of standardisation in SC 42. We conclude then by suggesting how this approach can be extended to enable similar comparisons with the use of concepts in documents being drafted by other SDO committees and by other bodies, including regulatory proposals, civic society policy proposals and guidelines developed by individual organisations.
Challenges to Building International Consensus on Governing Trustworthy AI
Since 2017 there has been an explosion in AI initiatives globally. As of February 2021, the Council of Europe’s tracker[2] has identified over 450 such initiatives world wide, primarily from national authorities, international organisations and the private sector. The most frequently discussed address subjects include privacy, human rights, transparency, responsibility, trust, accountability, freedom, fairness and diversity. Influential works such as the IEEE EAD [2], the EU’s High Level Expert Group on AI [4] and the OECD [5] often present these issues under the banner of ethical or trustworthy AI.
Scholars and think tanks have analysed this growing body of documents on ethical and trustworthy AI. One extensive survey identifies an apparent consensus on the importance of ethical principles of transparency, justice, non-maleficence, responsibility, and privacy, whereas other issues of sustainability, dignity, and solidarity in relation to labor impact and distribution garner far less attention across works [6]. Public authority works are identifying gaps in relation to the use of AI by governments and in weapon systems [7]. Private sector outputs have been criticized as instruments to reduce demand for government regulation [8], as potential barriers to new market entrants [9] and failing to address tensions between ethical and commercial imperatives within organisations [10]. A general criticism is a focus on individual rather than collective harms such as loss of social cohesion and harm to democratic systems [11].The required progression from approaches that propose broad principles, to specific and verifyiable practices that can be implemented by organisations and, where deemed necessary, regulated by legislation, implies a focus on governance and management of AI. Appropriate governance, management, and risk management measures can reinforce benefits and mitigate the ethical and societal risks of employing AI technology. Governance approaches can be characterized as [12]: market-based, resulting from value-chain partner pressures, including from consumers; self-organization, based on an organization’s internal policies; self-regulation based on industry wide agreement on norms and practices; and co-regulation based on industry compliance with government regulation and legislation. There have been some proposals for possible regulatory structure including: new national [13] and international [14] co-regulatory bodies and internal (self-regulatory) ethics boards that may help organizations implement best practice [15][16]. However, AI governance through co-regulation presents a number of major challenges [17]. These include: reaching stable consensus on what defines AI; widening access to AI skills and computing infrastructure obscuring developments from regulators; the diffusion of AI development over locations and jurisdictions globally; the emergence of impacts of an AI system only when assembled into a product or service; the opacity of modern subsymbolic machine learning methods and techniques, i.e. their unsuitability for clear human readable explanations; the potential for highly automated AI-driven systems to behave in unforeseeable ways that can escape the visibility or control of those responsible for them. More broadly, co-regulation is challenged by: the pacing problem, as AI technology develops faster than society’s ability to legislate for it; international cooperation needed for common standards being impeded by AI’s perceived role as a strategic economic or military resource; the perceived impediments of legislation to realising the competitive national economic and social benefits of AI; and the power asymmetry in AI capability being concentrated in digital platforms benefiting from network effects [9]. Over all types of works, a wide range of motivation have been identified [18], the incompatibility of some of which can further impede consensus on approaches to implementing trustworthy AI.
Nevertheless, there are multiple parallel standardisation activities ongoing internationally that are attempting to build some level of consensus, including the above mentioned IEEE P7000 and ISO/IEC JTC 1/SC 42 activities and several national activities[3]. This multiplicity of standards development may itself, however, contribute to inconsistencies and incompatibilities in how different organisations govern their AI activities. Reducing ambiguity in how different stakeholders in the AI value chain communicate with each other, and with society in general about their trustworthy AI practices is therefore critical to building trustworthiness of the resulting AI-based products and service. With both individual organisations and companies developing their own AI policies and legislation for AI regulation starting to be considered in major jurisdictions such as the EU, there is a need to support the ongoing mapping of concepts between these different parallel activities so that harmful or expensive inconsistencies can be identified early and hopefully resolved.
The following requirements for semantic interoperability between concepts developed by different bodies can therefore be identified and are depicted in Figure 1.:
- Consistency between documents being developed within the same standards family;
- Comparison between documents produced by parallel standards activities;
- Mapping between standards and regulatory/legislative proposal to determine the extent to which a standard can address regulatory requirements (US “safe harbor” or EU’s harmonized standards approach);
- Analysing the degree of compliance between a standard and an organisation’s AI policies and procedures, including their documentation outputs;
- Assessing organisational policies against regulations;
- Comparing different regulatory proposals internationally or comparing revisions to proposals in a single jurisdiction;
- Comparing AI policies published by different organisations
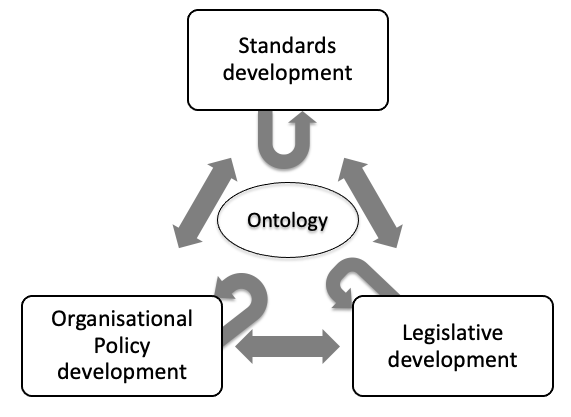
JTC1 Standards related to Trustworthy AI
Same as other ISO/IEC JTC 1 standardization activities, SC 42 places a strong emphasis on ensuring consistency with existing process and interoperability standards as well as reuse of existing terms and concepts to provide industry with a consistent body of applicable standards. SC 42 is therefore addressing AI-related gaps within existing standards, including those for management systems, risk management, governance of IT in organisations, IT systems and software quality. Rather than addressing AI ethics directly as a normative issue, SC 42 addresses the broader issues of trustworthy AI, with a technical report that sets out some of the core concepts and issues for standardisation related to Trustworthy AI (ISO/IEC 24028:2020)[19]. In this report, Trustworthiness is defined as the ability to meet stakeholders’ expectations in a verifiable way. When applied to AI, trustworthiness can be attributed to services, products, technology, data and information as well as organizations when considering their governance and management. This view considers trustworthy AI as realisable as part of a broader set of engineering, management, and governance process standards that can be employed together by organisations involved in AI and that can support mechanisms for conformity assessment including 3rd party certification and external oversight.
The Trustworthiness Working Group (WG 3) within SC 42 has a strong pipeline of pre-standardization and standardization activities. The roadmapping activities within the group are driven by gap analyses of prior art as well as current policy documents (including the IEEE EAD[2], HLEG [4], and OECD [5]). WG 3 builds on foundational terminology and high level life cycle notions elaborated within SC 42/WG 1 foundational deliverables ISO/IEC CD 22989 [20] on AI Concepts and Terminology and ISO/IEC CD 23053 [21] on a Framework for Artificial Intelligence (AI) Systems Using Machine Learning. WG 3 primarily looks at Trustworthiness high level characteristics and addresses them through elaboration of new project proposals of either pre-standardization informative deliverables, surveying the state of the art in an area (before proceeding to normative coverage at a later stage) or of normative deliverables.
The full fledged normative deliverable type within the ISO/IEC ecosystem is an International Standard (IS), however, not many areas in AI are mature enough to be addressed in international standards. This includes non-normative technical reports on current approaches to addressing societal and ethical aspects of AI [22] and bias in AI [23]. Thus WG 3 currently works only on three IS deliverables.
ISO/IEC CD 23894 Information Technology — Artificial Intelligence — Risk Management [24] is a specialization of ISO 31000 Risk Management [25]. This is an example of SC 42’s respect for prior art and application of existing frameworks such as quality, risk management, or management systems framework in the newly standardized area of AI and ML.
Another IS deliverable within the group is ISO/IEC WD 25059 Software engineering — Systems and software Quality Requirements and Evaluation (SQuaRE) — Quality model for AI-based systems [26]. This IS is an extension to the influential Systems and software Quality Requirements and Evaluation (SQuaRE) series owned by JTC 1/SC 7. Quality and trustworthiness are in a sense competing paradigms as they are looking at similar sets of high level characteristics such as robustness, reliability, safety, security, transparency, explainability etc. but the distinctive difference is in the need for quality stakeholders to take explicit part in actively defining quality requirements, while trustworthiness stakeholders don’t have to explicitly state their expectations in order to influence objective trustworthiness criteria. At any rate, the SQuaRE4AI standard sets a quality model that profiles the traditional quality and trustworthiness top level characteristics and their sub-characteristics for other normative deliverables in the area that are aiming at setting method and process requirements and recommendations.
The third IS in the making in WG 3 is ISO/IEC WD 24029-2 Artificial intelligence (AI) — Assessment of the robustness of neural networks — Part 2: Methodology for the use of formal methods [27]. This series aims to address the technical robustness pillar of AI trustworthiness, Part 2 specifically by looking at formally provable robustness and performance related properties of neural networks. While machine learning and neural networks in particular are an extremely active R&D field, the formal mathematical theory in which neural networks are based is well academically researched and stable. Therefore it is possible to benefit from known and provable properties of neural networks in current and upcoming industrial applications.
Technical Specification is a normative deliverable that has a less rigorous approval process, essentially there is only one round of national bodies approval for a TS compared to two distinct (and repeatable) stages for an IS approval. While it is easier to approve and publish a TS, a TS needs to be transformed into an IS or withdrawn 3 years after its publication. TS are sometimes called experimental standards. This type of deliverable is used in areas that are in urgent need of normative standardization as demonstrated by industry or societal demand, while the area to be standardized is still in flux from the research and development point of view. This is why WG 3 decided to ask SC 42 national bodies to approve development of ISO/IEC NP TS 6254 Information technology — Artificial intelligence — Objectives and methods for explainability of ML models and AI systems.
To develop an understanding of how these trustworthy AI standards relate to policies and processes defined by individual organisations and emerging as regulations in different jurisdictions requires an understanding of other aspects of AI standardisation under development in the other working groups of SC 42.
Working group 1 (WG 1) addresses foundational standards including the above-referenced AI Concepts and Terminology [20], which aims to provide consistency across the use of terms and concepts in other SC 42 documents and a Framework for Artificial Intelligence (AI) Systems Using Machine Learning [21] which reflects the central position of the machine learning area of AI in industry interoperability requirements.
Of importance to the mapping of AI standards to industry practice and regulation was the approval in August 2020, after a justification study, to develop an AI Management System (AIMS) Standard [28]. Management System Standards have a distinct role on the ISO ecosystem of standards types, as they provide the basis for certifying organisational processes. This provides a basis for organisations to demonstrate their conformance to specific standardised behaviour for management and related technical operations processes. Regulatory authorities also can make reference to such standards in specifying compliance regimes in complex technical domains. This allows authorities to manage the complexity and risk of technological change in regulations and to do so in a way that aligns with international industry and society consensus established through international standards. In contrast with industry consortia active in standardisation, standards produced by ISO and IEC are driven by national bodies (ISO and IEC members) who are typically mandated by their governments to represent a wider range of societal stakeholders than just industry. The overarching goal of these member organizations is to ease doing business according to the United Nations World Trade Organisation’s charter, as well as achieving United Nations’ sustainable development goals (SDGs).[4]
In recognition that big data plays a central role in the development of modern AI systems, Working group 2 (WG 2) of SC 42 has developed a series of big data standards. This includes a Big Data Reference Architecture (BDRA) [29] that provides a structured set of functional areas related to Big Data processing. Currently, WG 2 is developing a process management framework for big data analytics [30].
Finally, SC 42 also hosts and leads a joint working group (JWG 1) with JTC 1/SC 40 which addresses IT service management and IT governance in a specification for governance implications of the use of artificial intelligence by organizations [31]. This builds on the existing SC 40 standard providing guidance and principles for the effective, efficient, and acceptable governance of IT in an organisation [32].
Conceptual Modelling Approach
The formalisation of terminology is already a key element of international standards development [33], section 16. However, the use of ontologies to improve the consistency and coordination in international standards is not so well established, though it has been proposed [34]. There are some ambitious proposals to drive standards development together with implementation and verification from machine readable standards, with machine readable semantics of ontologies at its core [35], annex 11.4. The proposed application of ontologies to automated assistance in standards management and compliance, however, is outside the scope of this chapter.
ISO/IEC JTC 1/SC 32 has established requirements for ontologies to support semantic interoperability between information systems developed by different organisations and consortia across different application domains. These requirements address ontology definitions in a hierarchical manner with a top level ontology that provides core concepts that can be used in defining more specialised domain-specific ontologies [36]. Such ontologies are expressed through a combination of natural language definitions and a machine readable representation in a combination of description logic captured in the OWL2 language standard by the W3C [37] and Common Logic (CL) [38][b]. While CL is a full First Order Logic and therefore more expressive than OWL2, the latter offers the advantage of decidability by automated reasoners, therefore better supporting automated checking of specifications. For the top level ontology, the Basic Formal Ontology (BFO) is used [39]. To support the mapping between different domain models, the BFO adopts a realist design philosophy that aims to capture the objective reality in a domain rather than existing data representations. The BFO places a strong emphasis on representing temporal and spatial characteristics of concepts. For example, at the highest level it distinguishes between conceptual hierarchies for continuant entities that persist over time, and occurant entities that are time bound. Separate to its inclusion in ISO/IEC standardization’s use of ontologies, the BFO has been used for a set of biomedical ontologies [40] and for a collection of general common core ontologies addressing both horizontal concepts, known as mid level ontologies, e.g. on event, information, currency, and domain-specific ontologies such as spacecraft and ethnicity [41].
While the BFO provides a precise conceptual structure for modelling a wide range of concepts, its realist approach to ontology engineering implies a need for a centrally controlled programme of ontology development to ensure consistent use of top level concepts. While this would suit the objective of checking for and resolving consistency between a set of documents being developed under a common authority, e.g. within SC 42, the requirements for checking consistency between regulatory and organization policy drafts raises the needs to support a broad cohort of conceptual modellers who can operate without a common coordinating authority. An ontological approach that will be accessible for analysis and development by this wider range of conceptual modellers points therefore to demand less stringent conceptual modelling skills and is more accommodating to decentralised, domain-specific, and parallel development than BFO would enable.
The World Wide Web Consortium’s (W3C) Data Activity[5] builds on its earlier Semantic Web activity in developing industry standards for semantic interoperability that works to the scale and decentralised nature of the WWW. Grounded in the Resource Description Framework (RDF) [42] which allows an unlimited knowledge graph of nodes and links to exist as online resources on the WWW. Nodes and associations in this knowledge graph are typed according to ontologies, also known as data vocabularies, that can be developed independently and published to a distinct namespace on the WWW. This namespaced typing allows the free combination of types and associated conceptual knowledge from any published vocabulary. This has enabled the development and integration of a global network of over 1200 open and interlinked data sets, known as the linked open data cloud[6] [43]. Further logical axioms can be introduced to a vocabulary by including constructs from the Web Ontology Language (OWL) [37].The W3C restricts its ontology standardisation activities to those vocabularies that support the development, location, interlinking and use of datasets on the web and to application areas that are well aligned to decentralised data publishing, e.g. geospatial data. Organisations and consortia that develop vocabularies that address specific domains are free to publish them under their own name space, reusing aspects of other ontologies as needed. This highly decentralised approach therefore aligns well with the goal of promoting participation of those generating standards, organisational policies and regulations as well as those with an interest in how these documents develop and map to each other.
There is extensive research conducted on the use of ontologies in assessing compliance of organisational policies and practices with regulatory regulation [44]. Such assessment can be categorised as ex-ante compliance activities conducted before the regulated activity is performed or as ex-post compliance which is conducted after the regulated activities have occurred. The Provenance Ontology (PROV-O) is a W3C standard for the representation of provenance information using semantics provided by RDF and OWL [45]. PROV-O provides classes, properties, and restrictions to represent and interchange provenance information within different contexts. Its conceptual model is focussed on provenance of activities, the entities that those activities use and generate and the actors associated with those entities or to whom activities are attributed. PROV-O can be specialised to create new classes and properties to model provenance information for different applications and domains. This has been utilised to extend its conceptual model to specify ‘plans’ for ex-ante representations, scientific workflows, and domain-specific requirements for activities. An example of its usefulness is the representation of ex-ante and ex-post activities for evaluating compliance with EU’s General Data Protection Regulation [46], which is one of the more relevant extant regulations for AI. This existing capability, coupled with the decentralised conceptual modelling enables by the underlying RDF/OWL-based approach, leads us to adopt PROV-O, and its modelling of activities in particular, as the basis for conceptual modelling to support semantic interoperability between different sources of trustworthy AI concepts outline in section 2.
Ontology for Semantic Interoperability between Trustworthy AI Documents
The approach therefore taken in this chapter combines elements of BFO and PROV-O to provide a minimal and easy to understand ontology for mapping between different standard, regulations and organisational policies. Specifically we retain the distinction from BFO between continuant and occurant entities. For the continuant concept we adopt the PROV-O concept of Entity, which is defined as: a physical, digital, conceptual, or other kind of thing with some fixed aspects; entities may be real or imaginary. For the occurrent concept we adopt the PROV-O concept to Activity, which is defined as: something that occurs over a period of time and acts upon or with entities; it may include consuming, processing, transforming, modifying, relocating, using, or generating entities. We complement these core concepts of entity and activity with the PROV-O concept of Actor which is defined as: something that bears some form of responsibility for an activity taking place, for the existence of an entity, or for another agent's activity. BFO captures the organisational concept of a role as a subclass of continuant. However, the centrality of ethics and responsibility to the conceptual modelling of trustworthiness as well as for practical mapping to normative rules that are essential to standards, regulation and organisation policies demands that the concept of an Agent is made core alongside Entity and Activity. For the core relationships between these core concepts, we again draw from PROV-O relationships between these concepts, but recognising the benefit of the realist philosophy of BFO, these are phased in the present tense, rather than the past tense expression of PROV-O, which has a narrower focus on recording provenance i.e. what has existed and occurred. Therefore we introduce the following associations:
- Activity uses Entity: where usage is defined in PROV-O as the beginning of utilization of an Entity by an Activity, where before usage, the Activity had not begun to utilize this Entity and could not have been affected by the Entity.
- Activity generates Entity: where generation is defined by PROV-O as the completion of production of a new Entity by an Activity, where this Entity did not exist before generation and becomes available for usage after this generation. This therefore allows dependency chains to be assembled between activities.
- Entity attributedTo Agent: which is the ascribing of an Entity to an Agent, thereby conveying some sense of responsibility for the Entity to that Agent. This provides a short form for relating an Entity to an Agent when the Activity associated with the Actor that generates or uses the Entity is not specified.
- Activity associatedWith Agent: which PROV defines as an involvement by an Agent in an Activity.
- Activity informedBy Activity: which allows a dependency between two Activities to be defined without needing to specify the generation and use of an Entity between them.
- Agent actsOnBehalfOf Agent: which is used in PROV-O to express delegation, which is the assignment of authority and responsibility to an Agent to act as delegate or representative of another Agent. The delegation can be assigned to a specific Activity which implies that the delegate Agent therefore takes on a degree of responsibility for the Activity.
- Entity derivesFrom Entity: where PROV-O defines a derivation as a transformation of one Entity into another, an update of an Entity resulting in a new one, or the construction of a new Entity based on a pre-existing Entity. This relationship can be associated with a specific Activity, offering a similar chaining relationship to generatedBy and uses.
In addressing these core concepts and relationships, we express a scope related to the activities of organisations that relate to the trustworthiness of AI. Trustworthiness can be manifested through trustworthy characteristics of Entities that make up a product or service employing AI. Such AI trustworthiness characteristics can be associated with: the datasets used to train data-driven machine learning AI [47][48][49]; trained ML models [50][51] or AI-based product or services [52]. While some of these existing approaches share specific type of trustworthy characteristics for an entity, e.g. those related to bias in ML models or the processing of personal data, these differ according to the needs of the specific link in the AI value chain that is being served, i.e. is the organisation providing dataset, AI model or completed AI-based products and service to its customers. However, the common feature of the above use of trustworthy characteristics is that they are communicated between one actor in a value chain and another. As the SC 42 technical report on an Overview of AI trustworthiness [22] states, it is insufficient for one to simply refer to the ‘trustworthy AI’, but instead one must specify who trusts whom in what aspects of AI development and use. More specifically, the Actor that is providing an Entity to another Actor takes responsibility for ensuring that the trustworthy characteristics of the entity in question is exhibited, and not necessarily just to the Actor receiving them but to any interested parties.
Trustworthiness can also be manifested through the activities of an organisation providing entities that exhibit some trustworthy characteristics. The existing examples of approaches to expressing trustworthy characteristics of Entities referenced above, define many of those characteristics in terms of activities that were conducted to arrive at the characteristic, e.g. risk assessment.
To date, SC 42 has focussed on developing process-oriented standards, which is in line with the approach to developing Management Systems Standards (MSS) [53] annex SL. This approach is now being applied by SC 42 to develop a standard for an AI Management Systems (AIMS) [28]. A Management System is defined as a set of interrelated or interacting elements of an organization to establish policies and objectives and processes to achieve those objectives. An MSS aims to provide organisations with guidance on standards activities in a manner that may be subject to independent certification. Such certification can also play an important role in establishing trust in an organisation’s implementation of complex technical processes as part of a regulatory framework. Therefore the following concepts from [28] are added to the model as subclasses of Agent:
- Organisation: person or group of people that has its own functions with responsibilities, authorities and relationships to achieve its objectives [28]. It is the organisation which is the actor disclosing trustworthy characteristics.
- Stakeholder (used synonymously in [28] with an interested party): person or organization that can affect, be affected by, or perceive itself to be affected by a decision or activity, that are conducted by the Organisation implementing an AIMS. The Stakeholder is therefore the actor towards which any trustworthy characteristics is exhibited, with the aim of contributing to how that actor determines its level of trust in what Organisation claims possesses that characteristic.
Stakeholders can be internal or external to the Organisation. Therefore an Organisation will be involved in disclosing trustworthy characteristics to both internal and external stakeholder. Internal stakeholders include those with overall organisational governance responsibilities, i.e. the governing body, management and employees. Internal stakeholder also include those bound by Organisation by contracts, which would include shareholder and supply chain partners, i.e. providers, customer and individual consumers. External stakeholders such as regulators, government, supply chain partners, consumers, civic society, NGOs, the media and society in general.
As trustworthy characteristics are defined as a relationship between Organisations and Stakeholders, Entities being characterised are subclassed Assets. Assets are entities that represent some value to a stakeholder. For AI internal stakeholders, assets could include the data used to train or test an AI system, a trained AI model, a product or service that uses one or more AI systems, data consumed by an AI system in operation, software, computing and human resources used in training and operating an AI system [22].
Trustworthy characteristics may also relate to activities associated with Agents, which can be Organisations or Stakeholders. In considering the trustworthiness of an AIMS we would be focussed on the Activities conducted by the Organisation operating the AIMS. However, this subclassing of Agents also allows Activities with trustworthy characteristics also to be associated with Stakeholder, and in particular external Stakeholders, which allows complementary activities, e.g. in value chain partners or regulators to be modelled. While external Activities may not be an explicit part of an AIMS specification, they may be referenced in regulations or organisation policies which aim to clarify the interactions and share of responsibilities between Activities of the Organisation and those of such external Stakeholders.
The capture to different responsibilities within an Organisation operating an AIMS, additional semantics for the relationships between Activities (beyond dependencies via the use and generation of Entities and the dependsOn relationship) are required. Reference architectures and process model specifications often group activities into groups representing closely related or commonly co-occurant process. Activities are also sometime grouped into roles, which are a set of activities servicing some common purpose, the responsibility for which is often allocated together to a specific organisational unit. We therefore include a compositional relationship between Activities called partOf that allows for various for of Activity groups.
Within the context of an AIMS, and its mapping to regulations and policies of specific organisations, it is also important to capture some sense of different levels of responsibility and corresponding accountability. SC40 distinguishes between an organisation’s governance function and its management function as part of guidelines the governance of IT [32]. The governance function is responsible for understanding the external pressures in forming an organisation’s direction, strategy, objectives and policy in adopting IT, including customer, competitive, stakeholder expectation and regulatory pressures. The management function is then responsible for planning and achieving those objectives within the constraints of the strategy and policy. The governance function is structured into three activities, which are elaborated in the governance of IT implementation guide standards [54], Evaluate, Direct and Monitor. The evaluate activity addresses internal and external considerations in assessing plans and proposals from the management function. The direct activity sets direction through strategy and policy and assigns responsibilities for their realisation by the management function. The monitor activity assesses the performance of the realisation of governance direction on a regular basis, triggering further evaluation and direction if deficiencies are identified. Management functions are responsible for the control of technical operations activities, and may do so through delegation between appropriate levels of control. To capture the relationship between governance and management activities and between different levels of control between management activities, the following relationships between Activities in the ontology are defined: directedBy, evaluatedBy, monitoredBy and controlledBy.
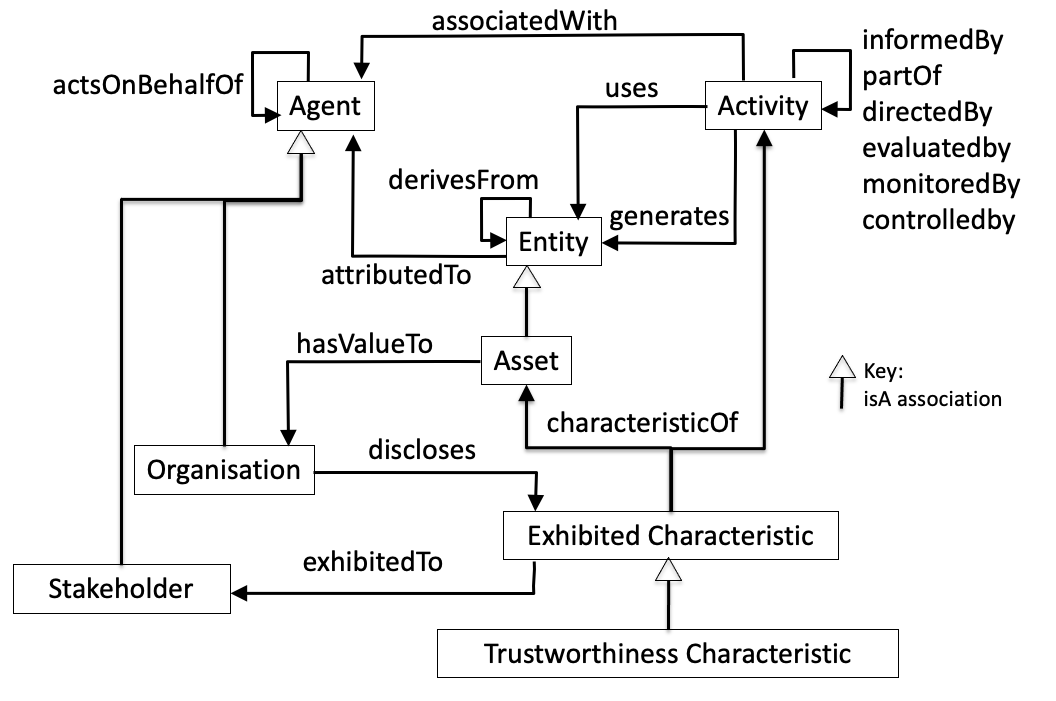
Figure 2, captures the above concepts and relationships into a core ontology to support the mapping of concepts between different emerging AI standards in SC 42 and between those standards and emerging organisational policies or governmental regulations. By restricting these concepts to a small core that is already established in existing standards, upon which some of the SC 42 standards are based, we anticipate this ontology will provide a robust basis for identifying the relationships between such concepts in different specifications. SC 42 has already identified characteristics that a trustworthy AI system or organisation involved in their implementation could exhibit [22]. These include technical characteristics such as reliability, robustness, verifiability, availability, resilience, quality, bias and robustness; stakeholder-related characteristics such as ethics, fairness and privacy; as well as management- and governance-related characteristics such as transparency, explainability, accountability and certification. However, the definition of many of these characteristics are still not yet well defined in relation to AI. By focussing on Activities, Entities and Agents we aim to identify mappings between concepts in different standards and therefore any gaps that can directly inform more consistent and comprehensive standards. In this way we hope to assist in the progression from broad statements of principles and areas of concern by the private sector, international bodies and governments, towards the develop of commonly understood process framework for the governance and management of Trustworthy AI, the ontology aims to do this is a way that accommodates the current range of definitions and interpretation of many of these characteristics and supports their convergence over time into concrete and internationally recognised governance and management processes and policies. In the following section we show how this process can be undertaken by using this ontology to map between the core anticipate AIMs and other relevant SC 42 specifications.
Modelling of AI Management System Activities
In 2020, SC 42 completed a justification study for a Management System Standard for AI. This was accepted in August and led to the initiation of a project to develop as AI Management System (AIMS) Standard [28], following the guidelines set out in the ISO/IEC Directive 1 [53]. All MSS should follow a consistent high level structure which includes common text and terminology as presented in Annex SL of this Directive. This is to allow different MSS addressing different horizontal and domain-specific areas to be integrated into the same overall management system within an organisation that implements these MSS. It is intended that MSS should be developed in a manner open to different stakeholders, including accreditation bodies, certification bodies, enterprises and the user community. The high level structure for MSS therefore provides a well defined source of concepts that are likely to be reflected in the MSS being developed for AI, which must also address management aspects of trustworthiness. The use of MSS for organisational process certification means it is also a suitable source of concepts that will be useful to track against those being developed for public and organisational policy and processes.
Table 1 presents a mapping of the MSS high level structure as a set of 17 AIMS activities, with each concept given an identifier, a label attribute, and a seeAlso attribute referencing the relevant section in the AI MSS draft, the numbering for which is mandated in the MSS high level structure. Each of these AIMS activities is attributed to either the Organisation (O) or Top Management (TM) as defined in the MSS high level structure, where the former attribution implies that activity spans governance and management levels. Relationship between these activities is captured by generates and uses attributes referencing 21 AIMS entities derived from the text in the MSS high level structure.
Activitity ID | rdf:label[c] | rdfs: seeAlso "42001-*" | attributedTo | generates (type Entity) | uses (type Entity, "aims*") |
aimsA1 | Understanding organisation and its concepts | 4.1 | O | aimsE1 - External and internal issues affecting AIMS outcomes | |
aimsA2 | Understanding stakeholder needs and expectation | 4.2 | O | aimsE2 - AIMS Stakeholders; aimsE3 - Stakeholder requirements | E1 |
aimsA3 | Determine AIMS scope | 4.3 | O | aimsE4 - AIMS Scope | E1, E2, E3 |
aimsA4 | Establish AIMS policy | 5.2 | TM | aimsE5- AI Policy | E1, E2, E3 |
aimsA5 | Assign roles, responsibilities and authorities | 5.3 | TM | aimsE6- Roles, responsibilities & authority assignments | E3, E4, E5 |
aimsA6 | Address risks and opportunities | 6.1 | O | aimsE7- AI risk management plan | E1, E2, E3 |
aimsA7 | Establish and plan to achieve AI objectives | 6.2 | O | aimsE8- AI objectives; aimsE9- Plan to achieve AI objectives | E3, E4, E5, E6 |
aimsA8 | Determine and allocate resources for AIMS | 7.1 | O | aimsE10- AI resource allocation | E9 |
aimsA9 | Determine and ensure competence of people affecting AI performance | 7.2 | O | aimsE11- AI competence plan | E9 |
aimsA10 | Promote awareness | 7.3 | O | aimsE12 - AI awareness plan | E3, E5, E6, E9 |
aimsA11 | Determine AIMS communication | 7.4 | O | aimsE13 - AI communication plan | E3, E9 |
aimsA12 | Plan and control AI processes | 8.1 | O | aimsE14 - AI operational process control plan | E6-E9 |
aimsA13 | Monitor, measure, analyse and evaluate AI | 9.1 | O | aimsE15 -AI & AIMS evaluation plan; aimsE16 - AI & AIMS evaluation results | E3-E8 |
aimsA14 | Internal audit | 9.2 | O | aimsE17 - AIMS audit plan; aimsE18 - AIMS audit results | E3-E6, E15, E16 |
aimsA15 | Undertake management review | 9.3 | TM | aimsE19 - AIMS change and improvement report | E1-E19 |
aimsA16 | Detect non conformance and take corrective action | 10.1 | O | aimsE20 - AIMS non conformity and corrective action report; aimsE21 - AIMS corrective action evaluation | E5, E8, E7 |
aimsA17 | AIMS Continual improvement | 10.2 | O | aimsE1 - aimsE15; aimsE17; aimsE20; aimsE21 | E1-E15 |
Table 1: Activity and Entities identified for an AIMS
Modelling of AIMs technical operation activities
The foundational standard on AI terms on concepts [20] entered its second committee draft ballot review towards the end of 2020 and is therefore still under development. However, section 6 of the draft does provide an outline of the lifecycle for an AI system made up of activities: inception; design and development, verification and validation; deployment; operation and monitoring; continuous validation; re-evaluation; and retirement. Table 2 shows a partially expanded breakdown of sub-activities that are part of these activities, exemplifying how the partOf attribute of these activities are used to identify the constituent activities. It also identifies certain sub-activities as also part of specific AIMS activities from table 1. Sub-activities under the Inception activity are identified as part of specific AIMS activities which would form part of the governance and management activities. The operations and monitoring activity (OM in table 2) of the AI lifecycle also contains some sub-activities that are part of AIMS activities, related to monitoring (aimsA13), communication (aimsA13) and risk (aimsA6). Further, all the AI lifecycle activities except Inception, are classified as technical operations activities, meaning within the AIMS they are directed by activity aimsA3 and aimsA4, evaluated by activities aimsA8 and aimsA9, monitored by activities aimsA13, aimsA14, aimsA15, aimsA16 and aimsA17 and controlled by activities aimsA12 (plan and control AI processes). The transitive nature of the partOf relationship therefore implies that the sub-activities of these AI lifecycle activities are also classed as technical operation activities with the same directedBy/evaluatedBy/monitoredBy/ controlledBy relationship to the corresponding AIMS activities. As AIMS activities can operate at different levels of management delegation, technical operations activities such as operations and monitoring (OM) therefore both play a part in conducting AIMS activities, but at a much narrower level of abstraction, as well as being subject to direction, evaluation and monitoring of other AIMS activities. It is notable that all the top layer activities in this lifecycle model, apart from retirement activities (RT) are part of the AIMS activity address risk (aimsA6).
ID 22989* | CD2 22989 - AI terms and concepts | partOf |
IC v | Inception | |
IC1 | Determine stakeholders' objectives | IC; aimsA1 |
IC2 | Determine stakeholders' requirements | IC; aimsA2 |
IC3 | Risk assessment and treatment planning | IC; aimsA6 |
IC4 | Policies and compliance planning | IC; aimsA4 |
DD ^* | Design and development | |
VV ^* | Verification and validation | |
DE ^* | Deployment | |
OM v* | Operation and monitoring | |
OM1 | Monitor AI system | OM; aimsA13 |
OM2 | Repair AI system | OM |
OM3 | Update AI system | OM |
OM4 | Support AI system users | OM; aimsA11 |
OM5 | OM5: Risk monitoring and review | OM; aimsA6 |
CV ^* | CV: Continuous validation | |
RE ^* | RE: Re-evaluation | |
RT ^* | RT:: Retirement |
Table 2: Partially expanded view of AI lifecycle activities and sub-activities from CD22989 []: v = constituent activities expanded, ^=constituent activities collapsed, *= AI technological operation activities
Though this lifecycle model from [20] is still a draft, it is being used in other SC 42 drafting activities, specifically the technical report on AI bias [23], which in section 9 breaks down bias treatment at each of the top levels activities of the lifecycle model, and a new work item on AI system life cycle processes to support their definition, control and improvement within an organisation or project [55].
As well supporting the mapping of AIMS activities into standards that explicitly define processes that we can model as activities, the ontology from Figure 2 can also be used to map AIMS into role-based frameworks. Specifically SC 42 Big Data Reference Architecture [29], which is structured into big data provider roles of: application provider, framework provider, service provider, data provider and consumer. These roles further are subdivided into sub-roles for which constituent activities are defined. This conforms with the notion of a role being a set of activities, so again this containment structure can be expressed as partOf relationship between the different levels of activity sets corresponds to those roles. All these are categories as technical operations activities in relation to the AIMS activities, though some also map to specific AIMs activities, with roles-based activities related to auditing (aimsA14) and requirements capture (aimsA7).
This mapping of activities reveals that while different standards developed under SC 42 are broadly consistent with the high level structure of AIMS, they vary in which areas of AIMS they focus on. While this may be arguably appropriate for the projects concerned, it does indicate that AIMS activities are not comprehensively mapping into other SC 42 standards as a matter of course. The ontology therefore provide a way of tracking these mapping and identifying gaps that may need to be addressed as the AIMS is specified or as changes to the other standards as they develop or are reviewed over time.
Modelling Stakeholders
The mapping of role-oriented activity processes from the Big Data Reference Architecture to AIMS activities also indicates how groupings of activities expressed as a role, e.g. Big Data Application Provider or Big Data Consumer, can also be used to model a stakeholder. The draft foundational terms and concepts standard from SC 42 [20] has similarly identified stakeholder in relation to similar types of value-chain role, but SC 42 has not yet attempted a more detailed characterisation of the activities that such stakeholders would undertake and which would therefore define such stakeholder roles.
However, many aspect of AI ethics and their impact on the trustworthiness of AI, relate to the impact on stakeholders who are not directly involved in the AI value chain, even as customers or consumers, e.g. pedestrians injured by an automated vehicle, local communities blighted by automated routing of commercial traffic through their neighbourhood, or people denied access to financial, health or social security services due to bias in algorithmic decision-making. In addition, such indirectly affected stakeholders may be difficult for organisations to identify and consult, so appropriate representation may be needed. Such representation could take the form of NGOs concerns with rights of certain groups, labour unions, professional bodies, local community groups, upto and including demoncratic forms of representation in the form of local and national governments. These issues are addressed however in the ISO Guidelines for Social Responsibility ISO26000 [56]. Its guidance is based on the fundamental practices of recognizing social responsibility within an organization and undertaking stakeholder identification and engagement. The guidance is based on the principles similar to those identified in the growing literature on AI ethics [6], including accountability, transparency and ethical behavior as well as respect for the rule of law, international norms of behavior and human rights. Social responsible guidance is provided in terms of 37 issues, each with suggested actions to address them and associated behavioral exceptions. These issues are each grouped under one of the following social responsibility core subjects: Human Rights; Labor practices; the Environment; Fair operating practices; Consumer issues; and Community involvement and development. The fact that these core subjects map onto different areas of legislation in many jurisdictions internally also eases the mapping of guidelines using this structure onto regulatory or other legal obligations that must be complied with by an organisation's management system. ISO26000 does not specifically address the use of AI, however these issues are sufficiently broad to provide a basis for mapping out specific ethical and societal issues associated with AI as shown through a comparison [57] with the normative language on trustworthy AI principles established in [2][4]and [5]. ISO already provides guidance in [58] on how to map principles and issues from ISO 26000 to the high level structure of MSS. SC 42 also recognises the importance of ISO 26000 guidance in handling stakeholder issues in the draft standards on AI governance [31] and AI risk management [24], with the draft technical report on ethical and societal concerns of AI [22] including a high level mapping of ISO26000 core issues onto AI risks and treatments.
Conclusion and Further Work
This chapter has highlighted the multiplicity of parallel activities being undertaken in developing international standards, regulations and individual organisational policies related to AI and its trustworthiness characteristics. The current lack of mappings between these activities presents the danger of a highly fragmented global landscape emerging in AI trustworthiness. This could present society, government and industry with competing standards, regulations and organisational practices that will then serve to undermine rather than build trust in AI. This chapter presents an overview of AI standardisation currently being undertaken in ISO/IEC JTC 1/SC 42 and identifies its project to define a AI management system standard as the starting point for establishing conceptual mapping between different initiatives. We propose a minimal, high level ontology for the support of conceptual mapping between different documents and show in the first instance how this can help map out the overlaps and gaps between SC 42 standards currently under development.
Much further work is required to develop and maintain a mapping between the ontological concepts and relationships identified from the emerging set of SC 42 AI standards and the emerging trustworthy AI regulations and policies from different organisations. The mapping of such standards to the ontology could be made publicly available in a findable, accessible, interoperable and reusable form, using linked open data principles [43], and updated as the referenced specifications evolve. This will assist in identifying gaps and inconsistencies between evolving drafts, especially in developing the AIMS standard [20]. The set of trustworthy AI characteristics could be captured in the ontology, based in the first instance on the AI engineering quality characteristic being developed in [square]. Similarly the ontology can be extended to express sets of AI risks and treatments so concepts developed in AI risk and bias will also be captured.
The use of this ontology-based approach for comparing the guidance between standards could also be applied between SC 42 and the largely orthogonal set of standards being developed under P7000. These include ethical design processes, transparency for autonomous systems, algorithmic bias, children, student and employee data governance, AI impact on human well-being, and trustworthiness rating for news sources.
Draft legislations for AI such as [9] will need to be analysed in terms of activities, actors, entities, characteristics and risks so that a mapping to the equivalent concepts from the SC 42 specifications family can be assembled and maintained. Similar analyses will be undertaken on publicly available policies from international bodies such as the EU High Level Expert Group on AI’s checklist for trustworthy AI [60] and the proposals emerging from the private sector for assigning trustworthiness declaration to products and services [47-51].
Acknowledgments
This work was conducted by the ADAPT Centre with support of SFI, by the European Union’s Horizon 2020 programme under the Marie Skłodowska-Curie Gran Grant Agreement No. 813497 and by the Irish Research Council Government of Ireland Postdoctoral Fellowship Grant GOIPD/2020/790. The ADAPT SFI Centre for Digital Content Technology is funded by Science Foundation Ireland through the SFI Research Centres Programme and is co-funded under the European Regional Development Fund (ERDF) through Grant # 13/RC/2106_P2.
Conflict of Interest
David Filip is the convener of ISO/IEC JTC 1/SC 42/WG3 and Dave Lewis is a contributor to several of the SC 42 standardisation projects covered in this chapter.
Notes/Thanks/Other declarations
Thanks to Delaram .Delaram Golpayegani and Arthit Suriyawongkul of the ADAPT Centre for their pertinent discussion and comments on the subject of this chapter.
References
- Adamson, G., Havens, J. C., & Chatila, R. “Designing a Value-Driven Future for Ethical Autonomous and Intelligent Systems”, Proceedings of the IEEE, 107(3), 518–525. 2019, DOI: 10.1109/JPROC.2018.2884923
- “Ethically Aligned Design – First Edition”, The IEEE Global Initiative
on Ethics of Autonomous and Intelligent Systems, IEEE, 2019. available at: https://standards.ieee.org/industry-connections/ec/ead-v1.html
- ISO GUIDE 82:2019 Guidelines for addressing sustainability in standards, Status available at: https://www.iso.org/standard/76561.html
- HLEG (2019) European Commission’s High Level Expert Group, “Ethics Guidelines for Trustworthy AI”, April 2019, https://ec.europa.eu/futurium/en/ai-alliance-consultation/guidelines
- OECD (2019) OECD/LEGAL/0449 “Recommendation of the Council on Artificial Intelligence”, https://legalinstruments.oecd.org/en/instruments/OECD-LEGAL-0449
- Jobin, A., Ienca, M. & Vayena, E. The global landscape of AI ethics guidelines. Nat Mach Intell 1, 389–399 (2019). DOI: 10.1038/s42256-019-0088-2
- Mapping regulatory proposals for artificial intelligence in Europe. Access Now, 2018 https://www.accessnow.org/mapping-regulatory-proposals-AI-in-EU
- Wagner, B., Ethics as an Escape from Regulation: From ethics-washing to ethics-shopping. In BEING PROFILED: COGITAS ERGO SUM: 10 Years of Profiling the European Citizen, edited by EMRE BAYAMLIOĞLU et al., Amsterdam University Press, Amsterdam, 2018, pp. 84–89. JSTOR, www.jstor.org/stable/j.ctvhrd092.18
- Calo, R. (2017). Artificial Intelligence Policy: A Roadmap. SSRN Electronic Journal, 1–28. DOI: 10.2139/ssrn.3015350
- Whittlestone, J., Nyrup, R., Alexandrova, A., Cave, S., The Role and Limits of Principles in AI Ethics: Towards a focus on tensions, in proc Artificial Intelligence, Ethics and Society, Honolulu, Hawaii, USA, Jan 2019
- Hagendorff, T. The Ethics of AI Ethics: An Evaluation of Guidelines. Minds & Machines 30, 99–120 (2020). DOI: 10.1007/s11023-020-09517-8
- Latzer, M., Hollnbuchner, K., Just, N., & Saurwein, F. (2016). The economics of algorithmic selection on the Internet. Handbook on the Economics of the Internet, (October 2014), pp 395–425. Retrieved from https://doi.org/10.4337/9780857939852.00028
- Tutt, A. (2016). An FDA for Algorithms. SSRN Electronic Journal, 83–123. https://doi.org/10.2139/ssrn.2747994
- Erdelyi, O.J. and Goldsmith, J., Regulating Artificial Intelligence: Proposal for a Global Solution (February 2, 2018). 2018 AAAI/ACM Conference on AI, Ethics, and Society (AIES '18), February 2--3, 2018, New Orleans, LA, USA. DOI: 10.1145/3278721.3278731, Available at SSRN: https://ssrn.com/abstract=3263992
- Calo, R. (2013). Consumer Subject Review Boards: A Thought Experiment. Stanford Law Review Online, 66(2002), 97.
- Polonetsky, J., Tene, O., & Jerome, J. (2015). Beyond the common rule: Ethical structures for data research in non-academic settings. Colo Tech L J, 13, 333–368.
- Scherer, M.U., (2016) Regulating Artificial Intelligence Systems: Risks, Challenges, Competencies and Strategies, Harvard Journal of Law & Technology, v29, no 2, Spring 2016
- Schiff, D., Borenstein, J., Biddle, J., Laas, K., "AI Ethics in the Public, Private, and NGO Sectors: A Review of a Global Document Collection," in IEEE Transactions on Technology and Society, doi: 10.1109/TTS.2021.3052127
- ISO/IEC TR 24028:2020 Information technology — Artificial intelligence — Overview of trustworthiness in artificial intelligence. 2020. Available from: https://www.iso.org/standard/77608.html
- ISO/IEC CD 22989.2, Artificial intelligence — Concepts and terminology, status available at: https://www.iso.org/standard/74296.html
- ISO/IEC CD 23053.2 Framework for Artificial Intelligence (AI) Systems Using Machine Learning (ML). Status available at: https://www.iso.org/standard/74438.html
- ISO/IEC AWI TR 24368 Information technology — Artificial intelligence — Overview of ethical and societal concerns. Status available at https://www.iso.org/standard/78507.html
- ISO/IEC DTR 24027 Information technology — Artificial Intelligence (AI) — Bias in AI systems and AI aided decision making. Status available at: https://www.iso.org/standard/77607.html
- ISO/IEC CD 23894 Information Technology — Artificial Intelligence — Risk Management. Status available at: https://www.iso.org/standard/77304.html
- ISO 31000:2018 Risk management — Guidelines. Available at: https://www.iso.org/standard/65694.html
- ISO/IEC AWI 25059 Software engineering — Systems and software Quality Requirements and Evaluation (SQuaRE) — Quality model for AI-based systems. Status available at: https://www.iso.org/standard/80655.html
- ISO/IEC AWI 24029-2 Artificial intelligence (AI) — Assessment of the robustness of neural networks — Part 2: Methodology for the use of formal methods. Status available at: https://www.iso.org/standard/79804.html
- ISO/IEC AWI 42001 Information Technology — Artificial intelligence — Management system. Status available at: https://www.iso.org/standard/81230.html
- ISO/IEC 20547-3:2020 Information technology — Big data reference architecture — Part 3: Reference architecture. Available from: https://www.iso.org/standard/71277.html
- ISO/IEC WD 24668:2020 Information Technology — Artificial intelligence — Process management framework for big data analytics. status available at: https://www.iso.org/standard/78368.html
- ISO/IEC CD 38507 Information technology — Governance of IT — Governance implications of the use of artificial intelligence by organizations. Status available at: https://www.iso.org/standard/56641.html
- ISO/IEC 38500:2015 Information technology — Governance of IT for the organization. Available at: https://www.iso.org/standard/62816.html
- ISO/IEC Directives, Part 2 Principles and rules for the structure and drafting of ISO and IEC documents, 8th Edition 2018, Available from: https://www.iso.org/directives-and-policies.html
- Narayanan, A., Lechevalier, D., Morris, K.C., Rachuri, S. Communicating standards through structured terminology, Computer Standards & Interfaces, Vol 40, 2015, Pages 34-41,ISSN 0920-5489, DOI: 10.1016/j.csi.2015.02.004
- Wahlster, W., Winterhalter,C., German Standardisation Roadmap for Artificial Intelligence, DIN & DKE, Nov 2020, Available from: https://www.din.de/resource/blob/772610/e96c34dd6b12900ea75b460538805349/normungsroadmap-en-data.pdf
- ISO/IEC DIS 21838-1:2019 Information Technology - Top-level ontologies (TLO) - Part 1: Requirements
- World Wide Web Consortium W3C Recommendation - OWL 2 Web Ontology Language Language Overview (2nd edition) available at: http://www.w3.org/TR/2012/REC-owl2-overview-20121211/
- ISO/IEC 24707:2018, Information Technology - Common Logic (CL): A framework for a family of logic-based languages, status available at: https://www.iso.org/standard/66249.html
- ISO/IEC: DIS 21838-2:2019 Information Technology - Top-level ontologies (TLO) - Part 2: Basic Formal Ontology (BFO)
- Smith B, Ashburner M, Rosse C, et al. The OBO Foundry: coordinated evolution of ontologies to support biomedical data integration. Nat Biotechnol. 2007;25(11):1251-1255. DOI:10.1038/nbt1346
- Rudnicki, R, An Overview of the Common Core Ontologies, CUBRC, Inc. Feb 2019, available from: https://www.nist.gov/system/files/documents/2019/05/30/nist-ai-rfi-cubrc_inc_004.pdf
- RDF 1.1 Primer,. Available: https://www.w3.org/TR/rdf11-primer/ (visited on 08/11/2019).
- Bizer, C., Heath, T., Berners-Lee,T., “Linked Data: The Story so Far”, Semantic Services, Interoperability and Web Applications: Emerging Concepts, pp. 205–227, 2011. DOI: 10/dh8v52. [Online]. Available: https://www.igi-global.com/chapter/linkeddata-story-far/55046 (visited on 09/17/2019).
- Fellmann, M., Zasada, A. State-of-the-Art of Business Process Compliance Approaches: A Survey, Proceedings of the 22nd European Conference on Information Systems (ECIS), Tel Aviv, June 2014
- Lebo, T., Sahoo, S., McGuinness, D., Belhajjame, K., Cheney, J., Corsar, D., Garijo, D., Soiland-Reyes, S., Zednik, S., Zhao, J., (2013). PROV-O: The PROV Ontology, http://www.w3.org/TR/2013/REC-prov-o-20130430/
- Pandit, H.J., . O’Sullivan, D., and D. Lewis, D., “Queryable Provenance Metadata For GDPR Compliance”, in Procedia Computer Science, ser. Proceedings of the 14th International Conference on Semantic Systems 10th – 13th of September 2018 Vienna, Austria, vol. 137, Jan. 1, 2018, pp. 262–268. DOI: 10/gfdc6r. [Online]. Available: http:/ /www.sciencedirect.com/science/article/pii/S1877050918316314 (visited on 10/18/2018).
- Gebru, T., Morgenstern, J., Vecchione, B., Vaughan, J.W., Wallach, H., Daumé, H., Crawford, K. “Data sheets for Datasets, in Proceedings of the 5th Workshop on Fairness, Accountability, and Transparency in Machine Learning”, Stockholm, Sweden, 2018.
- Bender, E., Freidman, B., “Data Statements for Natural Language Processing: Toward Mitigating System Bias and Enabling Better Science”, in Transactions of the Association for Computational Linguistics, vol. 6, pp. 587–604, 2018
- Holland, S., Hosny, A., Newman, S., Joseph, J., Kasia Chmielinski, K., (2018) The Dataset Nutrition Label: A Framework To Drive Higher Data Quality Standards. arXiv:1805.03677
- Arnold, M., et al., "FactSheets: Increasing trust in AI services through supplier's declarations of conformity," in IBM Journal of Research and Development, vol. 63, no. 4/5, pp. 6:1-6:13, 1 July-Sept. 2019, doi: 10.1147/JRD.2019.2942288.
- Mitchell, M., Simone Wu, S., Zaldivar, A., Barnes, P., Vasserman, L., Hutchinson, B., Spitzer, E., Raji, I.D., Gebru, T.,. 2019. Model Cards for Model Reporting. In Proceedings of the Conference on Fairness, Accountability, and Transparency (FAT* '19). Association for Computing Machinery, New York, NY, USA, 220–229. DOI:https://doi.org/10.1145/3287560.3287596
- Hallensleben et al, From Principles to Practice: An interdisciplinary framework to operationalise AI ethics, AI Ethics Impact Group, April 2020, Available from: https://www.ai-ethics-impact.org/en
- ISO/IEC Directives, Part 1, Consolidated ISO Supplement — Procedures specific to ISO, 11th edition 2020. Available from: https://www.iso.org/directives-and-policies.html
- ISO/IEC TS 38501:2015 Information technology — Governance of IT — Implementation guide, Available from: https://www.iso.org/standard/45263.html
- ISO/IEC WD 5338 Information technology — Artificial intelligence — AI system life cycle processes, Available from: https://www.iso.org/standard/81118.html
- ISO 26000:2010 Guidance on social responsibility, status available at: https://www.iso.org/standard/42546.html
- Lewis, D., Hogan, L., Filip, D., P. J. Wall, PJ., Global Challenges in the Standardization of Ethics for Trustworthy AI, Journal of ICT Standardization, vol 8, iss2, 2020, DOI: 10.13052/jicts2245-800X.823
- IWA 26:2017 Using ISO 26000:2010 in management systems, status available at: https://www.iso.org/standard/72669.html
- A9-0186/2020 REPORT with recommendations to the Commission on a framework of ethical aspects of artificial intelligence, robotics and related technologies (2020/2012(INL)), Committee on Legal Affairs, European Parliament, 8.10.2020
- HLEG European Commission’s High Level Expert Group, “The Assessment List for Trustworthy Artificial Intelligence (ALTAI) for self assessment”, July 2020,
[4] https://sdgs.un.org/goals
[a]TCD emails have long-term existence guarentees
[b]AFAIK no one does ontologies strictly/directly as CL, though RDF and OWL are considered definable subsets of CL.
[c]Column name can just be "label", then its easier for non-semweb readers (same for see also, generates, etc.)