AIRO: an Ontology for Representing AI Risks based on the Proposed EU AI Act and ISO Risk Management Standards
International Conference on Semantic Systems (SEMANTiCS)
✍ Delaram Golpayegani* , Harshvardhan J. Pandit , Dave Lewis
publication 🔓copies: harshp.com , TARA , zenodo
📦resources: repo , AIRO: an Ontology for Representing AI Risks based on the Proposed EU AI Act and ISO Risk Management Standards , specification
AI Risk Ontology (AIRO) for expressing information associated with high-risk AI systems based on the requirements of the proposed AI Act and ISO 31000 series of standards
Abstract The growing number of incidents caused by (mis)using Artificial Intelligence (AI) is a matter of concern for governments, organisations, and the public. To control the harmful impacts of AI, multiple efforts are being taken all around the world from guidelines promoting trustworthy development and use, to standards for managing risks and regulatory frameworks. Amongst these efforts, the first-ever AI regulation proposed by the European Commission, known as the AI Act, is prominent as it takes a risk-oriented approach towards regulating development and use of AI within systems. In this paper, we present the AI Risk Ontology (AIRO) for expressing information associated with high-risk AI systems based on the requirements of the proposed AI Act and ISO 31000 series of standards. AIRO assists stakeholders in determining `high-risk' AI systems, maintaining and documenting risk information, performing impact assessments, and achieving conformity with AI regulations. To show its usefulness, we model existing real-world use-cases from the AIAAIC repository of AI-related risks, determine whether they are high-risk, and produce documentation for the EU's proposed AI Act.
AI ,Ontology ,Semantic Web ,Risk ,Risk Management ,AI Act ,ISO
Introduction
The adoption of AI has brought many benefits to individuals, communities, industries, businesses, and society. However, use of AI systems can involve critical risks as shown by multiple cases where AI has negatively impacted its stakeholders by producing biased outcomes, violating privacy, causing psychological harm, facilitating mass surveillance, and posing environmental hazards [1], [2]. The growing number of incidents caused by (mis)using AI is a matter of concern for governments, organisations, and the public. With the rapid progression of AI technologies and the wide adoption of innovative AI solutions, new forms of risk emerge quickly, which in turn adds to the uncertainties of already complex AI development and deployment processes. According to ISO risk management standards, risk management practices aim to manage uncertainties, in this case regarding AI systems and their risks, by adopting a risk management system for identification, analysis, evaluation, and treatment of risks [3].
To guide and in some cases mandate organisations in managing risk of harms associated with AI systems, multiple efforts are currently underway across the globe. These activities aim to provide recommendations on development and use of AI systems, and consist of creating ethical and trustworthy AI guidelines [4], developing AI-specific standards such as the AI risk management standard [5], and establishing AI regulatory frameworks - prominently the EU’s AI Act proposal (hereafter the AI Act) [6].
The AI Act aims to avoid the harmful impacts of AI on critical areas such as health, safety, and fundamental rights by setting down obligations which are proportionate to the type and severity of risk posed by the system. It distinguishes specific areas and the application of AI within them that constitutes ‘high-risk’ and has additional obligations (Art. 6) that require providers of high-risk AI systems to identify and document risks associated with AI systems at all stages of development and deployment (Art. 9).
Existing risk management practises consist of maintaining, querying, and sharing information associated with risks for compliance checking, demonstrating accountability, and building trust. Maintaining information about risks for AI systems is a complex task given the rapid pace with which the field progresses, as well as the complexities involved in its lifecycle and data governance processes where several entities are involved and need to share information for risk assessments. In turn, investigations based on this information are difficult to perform which makes their auditing and assessment of compliance a challenge for organisations and authorities. To address some of these issues, the AI Act relies on creation of standards that alleviate some of the compliance related obligations and tasks (Art. 40).
In this paper, we propose an approach regarding the information required to be maintained and used for the AI Act’s compliance and conformance by utilising open data specifications for documenting risks and performing AI risk assessment activities. Such data specifications utilise interoperable machine-readable formats to enable automation in information management, querying, and verification for self-assessment and third-party conformity assessments. Additionally, they enable automated tools for supporting AI risk management that can both import and export information meant to be shared with stakeholders - such as AI users, providers, and authorities.
The paper explores the following questions: (RQ1) What is the information required to determine whether an AI system is ‘high-risk’ as per the AI Act? (RQ2) What information must be maintained regarding risk and impacts of high-risk AI systems according to the AI Act and ISO risk management standards? (RQ3) To what extent can semantic web technologies assist with representing information and generating documentation for high-risk AI systems required by the AI Act?
To address RQ1 and RQ2, in Section 3.2, we analyse the AI Act and ISO 31000 risk management series of standards to identify information requirements associated with AI risks. To address RQ3, we create the AI Risk Ontology (AIRO), described in Section 3.3, and demonstrate its application in identification of high-risk AI systems and generating documentation through analysis and representation of real-world use-cases in Section 4.
State of the Art
AI Risk Management Standards
The ISO 31000 family of standards support risk management in organisations by providing principles, guidelines, and activities. ISO 31000:2018 Risk management – Guidelines [3] is the main standard that provides generic principles, framework, and processes for managing risks faced by organisations throughout their lifecycle. Another member of this family is ISO 31073:2022 Risk management — Vocabulary [7] which provides a list of generic concepts in risk management and their definitions to promote a shared understanding among different business units and organisations.
There is ongoing work within ISO to further apply these risk standards within the domains and processes associated with AI. In particular, ISO/IEC 23894 Information technology — Artificial intelligence — Risk management [5] specifically addresses risk management within AI systems. Efforts are also underway to provide agreements on a vocabulary of relevant AI concepts (ISO/IEC 22989 [8]) and addressing ethical and societal concerns (ISO/IEC TR 24368 [9]). These are intended to be utilised alongside recently published standards regarding AI, such as those relating to trustworthiness (ISO/IEC TR 24028:2020 [10]), and bias and decision making (ISO/IEC TR 24027:2021[11]).
AI Risk Taxonomies
There is a growing body of literature on discovering types of risk stemming from AI techniques and algorithms. For example, a taxonomy of AI risk sources, proposed in [12], classifies the sources that impact AI trustworthiness into two categories: sources which deal with ethical aspects and the ones that deal with reliability and robustness of the system. The US National Institute of Standards and Technology (NIST) [13] has developed an AI risk management framework which includes a taxonomy of the characteristics that should be taken into account when dealing with risks. The taxonomy identifies three categories of risk sources associated with AI systems, namely sources related technical design attributes such as accuracy, sources related to the way the system is perceived e.g. transparency, and sources associated with principles mentioned in trustworthy AI guidelines e.g. equity. The framework also identifies three types of harmful impacts: harm to people, harm to an organisation/enterprise, and harm to a system.
Andrade and Kontschieder [14] developed a taxonomy of potential harms associated with machine learning applications and automated decision-making systems. The taxonomy identifies the root cause of the harms, their effects, the impacted values, and technical and organisational measures needed for mitigating the harms. Roselli et al. [15] proposed a taxonomy of AI bias sources and mitigation measures, which classifies AI bias into three categories based on the source: bias that arises from translating business goals to system implementation, bias stemmed from training datasets, and bias that is present in individual input samples.
The mentioned studies provide taxonomies without formally modelling the relationships that exist between concepts, e.g. the relation between risk and its controls that indicates which controls are suitable or effective to mitigate the risk. An ontology that expresses the semantic relations between risk concepts enables reasoning over risk information and exploring patterns in the risk management process. This paper goes further than defining a hierarchy of concepts and proposes an ontology for AI risk. The identified concepts and proposed classifications in resources such as the aforementioned studies can be used to populate the AI risk ontology.
Risk Models and Ontologies
There are attempts to provide a general model of risk such as the common ontology of value and risk [16] which describes risk by associating it to the concept of value and the ontology presented in [17] which models the core concepts and relations in ISO/IEC 27005 standard for infrastructure security risk management.
There are also several studies where ontologies were developed to facilitate risk management in different areas such as construction and health. For instance, Masso et al. [18] developed SRMO (Software Risk Management Ontology) based on widely-used risk management standards and guidelines to address ambiguity and inconsistency of risk terminologies. Hayes [19] created a risk ontology to represent the risk associated with online disclosure of personal information. A key feature of this ontology is separation of consequence of risk from harm. McKenna et al. [20] implemented the Access Knowledge Risk (ARK) platform which employs SKOS data models to enable risk analysis, risk evidence collection, and risk data integration in socio-technical systems.
To the best of our knowledge, there is no ontology available for expressing fundamental risk concepts based on ISO 31000 series of standard, nor one specific to AI risks. Our future ambition is to investigate the state of the art in the areas of (AI) risk modelling as the literature advances and systematically compare our work with the recent advances in an iterative manner.
AIRO Development
Given the lack of readily available semantic ontologies regarding risk management and AI systems, answering RQ3 regarding use of semantic web technologies necessitated creation of an ontology to represent risks associated with AI systems based on ISO risk management standards. The AI Risk Ontology (AIRO) provides a formal representation of AI systems as per the requirements of the AI Act with the risk and impacts being represented based on ISO 31000 family of standards. It is the first step in identifying and demonstrating the extent of semantic web technologies in enabling automation of risk documentation, querying for legal compliance checking, and facilitating risk information sharing for the AI Act and other future regulations.
Methodology
The development of AIRO followed the “Ontology Development 101” guideline provided by Noy and McGuinness [21] and the Linked Open Terms (LOT) methodology [22]. The steps followed for creating AIRO are as follows:
Ontology requirements specification: The requirements regarding identification of high-risk AI systems and generating technical documentation are extracted from the AI Act and materialised as competency questions.
Ontology implementation: To build the ontology we first identify core risk concepts and relations from ISO 31000 series of standards. The top-level AI concepts are derived from the AI Act. Then, the Act and ISO/IEC FDIS 22989 Information technology — Artificial intelligence — Artificial intelligence concepts and terminology [8], which provides a uniform reference vocabulary regarding AI concepts and terminology, are used for further expanding the core concepts.
Ontology evaluation: To ensure that AIRO fulfils the requirements identified in the first step, the ontology is evaluated against the competency questions and its applicability is evaluated by modelling example use-cases from the AIAAIC repository [2]. The quality of the ontology is ensured by following Semantic Web best practices guidelines, including W3C Best Practice Recipes for Publishing RDF Vocabularies1 and the OntOlogy Pitfall Scanner (OOPS!) [23].
Ontology publication: The documentation is created using WIDOCO [24] - a tool for generating HTML documents from ontology metadata. AIRO is available online at https://w3id.org/AIRO under the CC BY 4.0 license.
Ontology maintenance: Since the proposed AI Act is subject to change, requirements and concepts derived from it will need to be revised as newer versions are published. Additionally, relevant documents including trustworthy AI guidelines and AI incident repositories e.g. AIAAIC, will also influence the design through concepts such as types of AI and known impacts. This leads to an iterative process for updating the ontology, with appropriate documentation of changes.
AIRO Requirements
The purpose of AIRO is to express AI risks to enable organisations (i) determine whether their AI systems are ‘high-risk’ as per Annex III of the AI Act and (ii) generate the technical documentation required for conformity to the AI Act.
Describing High-Risk AI Systems
The EU’s proposed AI Act aims to regulate the development, deployment, and use of AI systems with the purpose of eliminating harmful impacts of AI on health, safety, and fundamental rights. At the heart of the Act there is a four-level risk pyramid that classifies AI systems into the following categories where the level of risk corresponds to the strictness of rules and obligations imposed: 1) prohibited AI systems, 2) high-risk AI systems, 3) AI systems with limited risk, 4) AI systems with minimal risk.
According to the AI Act, AI systems are software systems that are developed using at least one of the three types of techniques and approaches listed in Annex I namely, machine learning, logic- and knowledge-based, and statistical approaches. High-risk AI systems are either (i) a product or safety component of a product, for example medical devices, as legislated by existing regulations listed in Annex II; or (ii) systems that are intended to be used in specific domains and purposes as mentioned in Annex III.
A major part of the AI Act is dedicated to the requirements of
high-risk AI systems and the obligations for providers and users of
these systems. To understand their legal obligations regarding the
development and use of AI systems, providers need to identify whether
the system falls into the category of high-risk. To facilitate this
process, we analysed the requirements of the AI Act, in particular the
list of high-risk systems in Annex III, and identified the specific
concepts whose combinations determine whether the AI system is
considered high-risk; for example, according to Annex III 6(d), use of
AI in the domain of law enforcement (Domain
) by law
enforcement authorities (AI User
) for evaluation of the
reliability of evidence (Purpose
) in the course of
investigation or prosecution of criminal offences
(Environment Of Use
) is high-risk. These are listed in
Table 1 in
the form of: competency questions, concepts, and relation with AI
system.
Competency question | Concept | Relation |
---|---|---|
What techniques are utilised in the system? | AITechnique |
usesTechnique |
What domain is the system intended to be used in? | Domain |
isAppliedWithinDo-main |
What is the intended purpose of the system? | Purpose |
hasPurpose |
What is the application of the system? | Application |
hasApplication |
Who is the intended user of the system? | AIUser |
isUsedBy |
Who is the subject of the system? | AISubject |
affects |
In which environment is the system used? | EnvironmentOfUse |
isUsedInEnvironment |
Technical Documentation
To conform to the AI Act, high-risk AI systems need to fulfil the requirements laid out in Title III, Chapter 2. One of the key obligations is implementing a risk management system to continuously identify, evaluate, and mitigate risks throughout the system’s entire lifecycle (Art. 9). To demonstrate conformity to authorities, the providers of high-risk systems need to create a technical documentation (Art. 11) containing information listed in Annex IV. In addition, providers have to identify the information needed to be registered in the EU public database (Art. 60) and provided to the users (Art. 13) [25].
To assist with this process, we identified the information required to be provided as the technical documentation for an AI system as per AI Act Annex IV, with relevant concepts and relations as presented in Table 2. Recording the sources from which the ontology’s requirements are identified is helpful in the maintenance process where AIRO should be updated with regard to the amendments that will be applied to the AI Act.
Annex IV Clause | Required information | Domain | Relation | Range |
---|---|---|---|---|
1(a) | System’s intended purpose | AISystem | hasPurpose | Purpose |
System’s developers | AISystem | isDevelopedBy | AIDeveloper | |
System’s date | AISystem | dcterms:date | ||
System’s version | AISystem | hasVersion | Version | |
1(c) | Versions of relevant software or firmware | System/ Component | hasVersion | Version |
1(d) | Forms in which AI system is placed on the market or put into service | AISystem | isUsedInFormOf | AISystemForm |
1(e) | Hardware on which the AI system run | AISystem | hasExecutionEnvironment | AIHardware |
1(f) | Internal layout of the product which the system is part of | AISystem | hasDocumentation | Blueprint |
1(g) | Instruction of use for the user | AISystem | hasDocumentation | InstructionOfUse |
Installation instructions | AISystem | hasDocumentation | InstallationInstruction | |
2(a) | third party tools used | AISystem | hasComponent | Tool |
Pre-trained system used | AISystem | hasComponent | Pre-trainedSystem | |
2(b) | Design specifications of the system | AISystem | hasDocumentation | SystemDesignSpecification |
2(c) | The system architecture | AISystem | hasDocumenatation | SystemArchitecture |
2(d) | Data requirements | Data | hasDocumentation | Datasheet |
2(e) | Human oversight measures | HumanOversightMeasure | modifiesEvent | Event |
2(g) | Testing data | AISystem | hasComponent | TestingData |
Validation data | AISystem | hasComponent | ValidationData | |
Characteristics of data | Data | hasDocumentation | Datasheet | |
Metrics used to measure accuracy/ robustness/ cybersecurity | Accuracy/ Robustness/ CybersecurityMertic | isUsedToMeasure | AISystemAccuracy/ Robustness/ Cybersecurity | |
Discriminatory impacts of the system | Consequence | hasImpact | Impact | |
Test log | AISystem | hasDocumentation | TestLog | |
Test report | AISystem | hasDocumentation | TestReport | |
3 | Expected level of accuracy | AISystem | hasExpectedAccuray | AISystemAccuracy |
Foreseeable unintended outcomes of the risk | Risk | hasConsequence | Consequence | |
Sources of the risk | RiskSource | isRiskSourceFor | Risk | |
Human oversight measures | HumanOversightMeasure | modifiesEvent | Event | |
Technical measures | TechnicalMeasure | modifiesEvent | Event | |
Specification of input data | InputData | hasDocumentation | Datasheet | |
4 | Risks associated with the AI system | AISystem | hasRisk | Risk |
Sources of the risk | RiskSource | isRiskSourceFor | Risk | |
Consequences of the risk | Risk | hasConsequence | Consequence | |
Harmful impacts of the risk | Consequence | hasImpact | Impact | |
Probability of risk source/ risk/ consequence /impact | RiskSource/ Risk/ Consequence/ Impact | hasLikelihood | Likelihood | |
Severity of consequence/ impact | Consequence/ Impact | hasSeverity | Severity | |
Impacted stakeholders | Impact | hasImpactOnAISubject | AISubject | |
Impacted area | Impact | hasImpactOnArea | AreaOfImpact | |
Risk management measures applied | Control | modifiesEvent | Event | |
6 | Standard applied | AISystem | usesStandard | Standard |
Harmonised standards applied | AISystem | usesStandard | HarmonisedStandard | |
Technical specifications applied | AISystem | usesTechnicalSpecification | TechnicalSpecification | |
7 | EU declaration of conformity | AISystem | hasDocumentation | EUDeclarationOfConform-ity |
8 | Post-market monitoring system | AISystem | hasPostmarketMonitoring System | PostmarketMonitoringSys-tem |
Description of the post-market system that evaluates the performance | PostmarketMonitoringSy-stem | dcterms:description |
AIRO Overview
AIRO’s core concepts and relations are illustrated in Figure 1.
The upper half shows the main concepts required for describing an
AI System
(green boxes), and the lower half represents key
concepts for expressing Risk
(yellow boxes). The relation
hasRisk
links these two halves by connecting risk to either
an AI system or a component of the system.
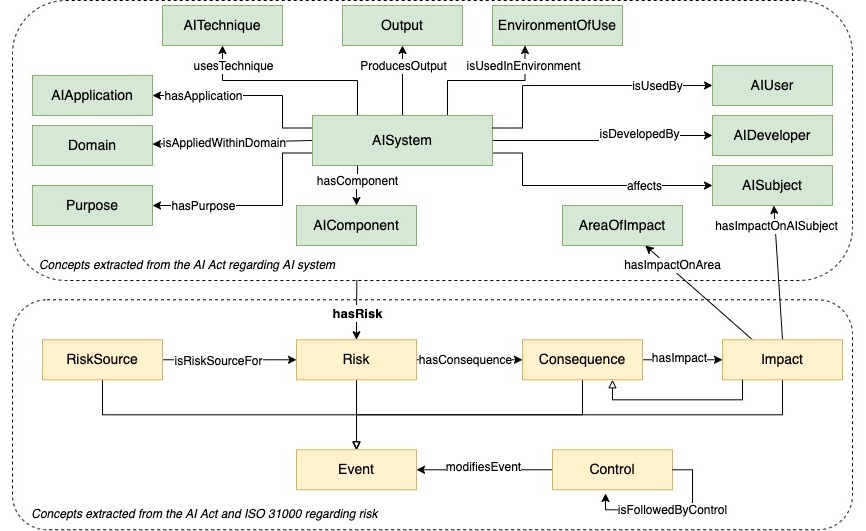
The core concepts related to an AI System
are: (1) the
intended Purpose
of the system, (2) the Domain
the AI system is supposed to be used in, (3) the
AI Application
of the system, (4) the
Environment Of Use
which specifies the environment the
system is designed to be used in, e.g. publicly accessible spaces, (5)
the AI Technique(s)
utilised by the system such as knowledge-based, machine learning, and statistical approaches, (6)
Output(s)
the system generates and (7) the system’s
incorporating AI Component(s)
. Furthermore, the key
stakeholders in the AI value chain are modelled including (8)
AI Users
who utilise the system, (9)
AI Developers
that develop(ed) the AI system, and (10)
AI Subjects
that are impacted by the system including
individuals, groups, and organisations. To specify the area that is
impacted by the system the concept of (11) Area Of Impact
is defined.
The key risk concepts in AIRO are: (1) Risk Source
,
indicates an event that has the potential to give rise to risks, (2)
Consequence
, indicates an outcome of risks, (3)
Impact
, represents an effect of consequences on
AI Subject(s)
, and (4) Control
, indicates a
measure that is applied to detect, mitigate, or eliminate risks. ISO
31000 sees risk as being both an opportunity and a threat. However, in
the context of the AI Act the concept of risk, and therefore its
consequence and impact, refers to the risk of harm. To reflect this,
AIRO only refers to risks in the context of harms. AIRO also
distinguishes between Consequence
and Impact
to indicate consequence as direct outcomes which may or may not involve
individuals, which can then lead to an impact (harm) to some AI
subjects. Risks, consequences, and impacts can be addressed using
Control
that can relate to detection, mitigation, and
elimination.
To further expand AIRO, the top-level concepts are populated by the
classes obtained from the AI Act and ISO/IEC 22989. Then, the classes
are categorised using a bottom-up approach. To give an example, the AI
Act refers to some of the potential purposes of using AI, such as
dispatching emergency services, generating video content (using
deepfake), monitoring employees’ behaviour, and assessing tests. After
identifying sub-classes of Purpose, they
are classified into more general categories. In this case, we identified
six high-level classes for Purpose
namely, Generating Content, Knowledge Reasoning, Making Decision, Making Prediction, Monitoring, and Producing Recommendation. The current version
of AIRO incorporates 45 object properties and 276 classes, including 13
AI Techniques
, 76 Purposes
, 47
Risk Sources
, 18 Consequences
, 7
Areas of Impact
, and 18 Controls
.
Applying AIRO by Modelling Real-World Use-Cases
The AI and Algorithmic Incidents and Controversies (AIAAIC) is an ongoing effort to document and analyse AI-related problematic incidents. As of July 2022, it has over 850 incidents collected from news articles, reports, and other sources. Here, we utilise two scenarios from this repository, selected based on availability of detailed information regarding AI system in use and topicality, and manually represent them using AIRO, with potential for automation in future. We then evaluate and demonstrate how AIRO can be used to query relevant information, identify missing concepts, and generate technical documentation - as per the AI Act. RDF representations for both are available online2.
Use-case 1: Uber’s Real-time ID Check System
This use-case3 describes an instance where Uber used a facial recognition identification system, known as the Real Time ID (RTID), to ensure that the driver’s account is not used by anyone other than the registered Uber driver. If the system failed to recognise a person for two consecutive times, the driver’s contract would be terminated and their driver and vehicle licenses would be revoked. Multiple incidents where the system failed to verify drivers of BAME (Black, Asian, Minority Ethnic) background proved that the use of the facial recognition system involved risks of inaccuracy which could have lead to unfair dismissal of drivers. Figure 2 illustrates how AIRO is used in modelling the use-case described.
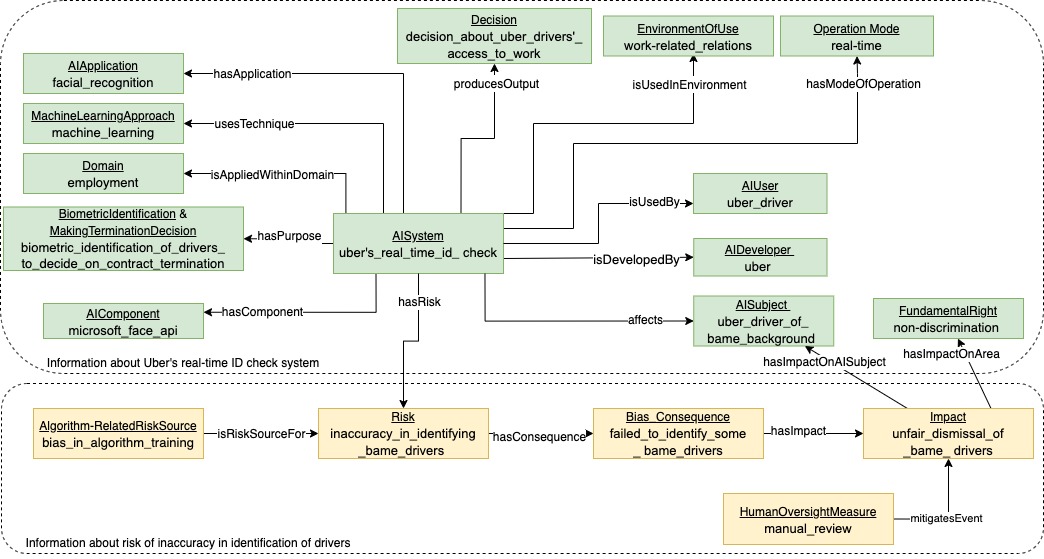
Use-case 2: VioGén Domestic Violence System
This use-case4 describes the VioGén Domestic Violence System that was used by the Spanish law enforcement agencies to assess the likelihood of a victim of gender violence to be assaulted by the same perpetrator again, which is used for determining the victim’s eligibility for police protection [26].
Its use of statistical models to predict the risk faced by a victim raise questions regarding the accuracy of its predictions since these would be highly dependent on the quality of data fed into the models. The input data was generated based on a questionnaire answered by victims who filed a report. The ambiguity of questions and timing of questionnaire could have lead to inaccurate or biased predictions, and if the score was not modified by police officers - the victim would not required protection. To control this risk, police officers were granted the power to increase the risk score calculated by the system. However, according to [27], in most cases the officers trusted the system’s scoring despite warning signs, which led to “automation bias” i.e. over-reliance on the system’s outcomes. Figure 3 shows the representation of this use-case using AIRO.
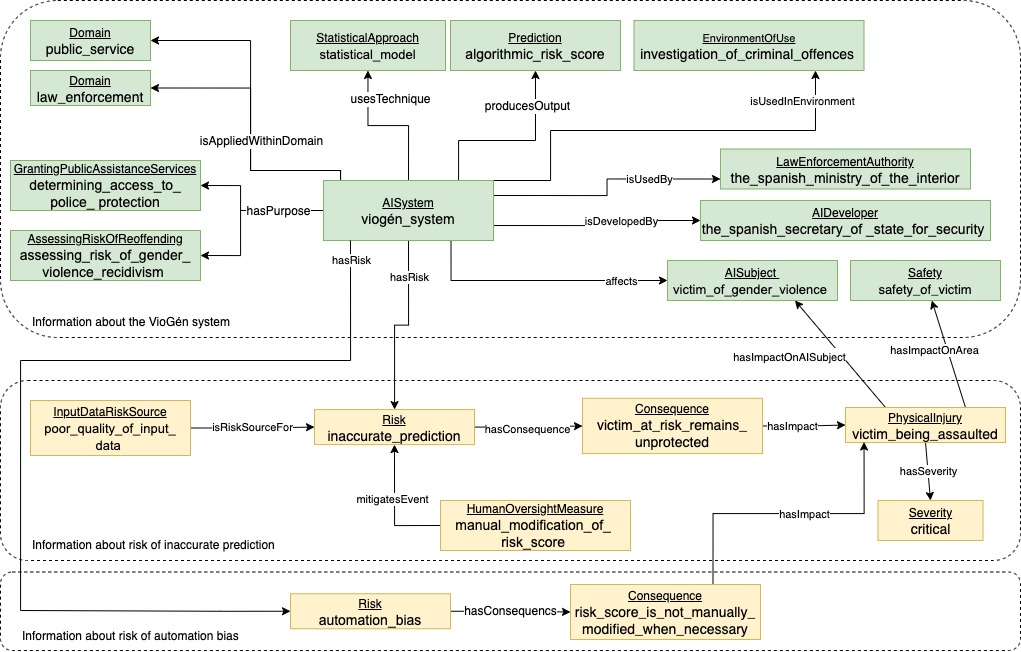
Identification of High-risk AI Systems
To assist with determination of whether the system would be
considered a high-risk AI system under the AI Act, the concepts
presented in Table.1
need to be retrieved for the use-case and compared against the specific
criteria described in Annex III. This can be achieved through several
means: such as using a SPARQL ASK
query, SHACL shapes, or
any other rule-based mechanism.
For demonstration, we first utilise a SPARQL query, depicted in Listing 1, to list the concepts necessary to determine whether the system is high-risk (see Table 3). It is worth noting that one of the contributions of this paper is translating the high-risk conditions specified in Annex III of the AI Act into 7 concepts which can be retrieved using the SPARQL query depicted in Listing 1. A manual inspection of the use-cases and query results shows that both systems would be considered as high-risk under the AI Act. Uber’s system falls within the category of high-risk since it was employed for the purpose of biometric identification of natural persons (Annex III,1-a) and for making decisions on termination of work-related relationships (Annex III, 4-b). VioGén system is considered a high-risk AI system as it is employed by law enforcement authorities as means for predicting the risk of gender violence recidivism (Annex III, 6-a) that in turn is used for determining access to public services, i.e. police protection (Annex III, 5-a).
Listing 1: SPARQL query retrieving information for determining high-risk AI systems
PREFIX airo: <https://w3id.org/AIRO#>
SELECT ?system ?technique ?domain ?purpose
?application ?user ?subject ?environment
WHERE {
?system a airo:AISystem ;
airo:usesTechnique ?technique ;
airo:isUsedWithinDomain ?domain ;
airo:hasPurpose ?purpose ;
airo:hasApplication ?application ;
airo:isUsedBy ?user ;
airo:affects ?subject ;
airo:isUsedInEnvironment ?environment . }
AIRO concept | Uber’s Real-time ID Check | VioGén system |
---|---|---|
AISystem | uber’s_real_time_id_check | viogén_system |
AITechnique | machine_learning | statistical_model |
Purpose | biometric_identification_of_drivers_to _decide_on_contract_termination | determining_access_to_police_protection & assessing_risk_of_gender_violence _recidivism |
Domain | employment | law_enforcement & public_service |
AIApplication | facial_recognition | profiling |
AIUser | uber_driver | the_spanish_ministry_of_the_interior |
AISubject | uber_driver_of_bame_background | victim_of_gender_violence |
EnvironmentOfUse | work_relate_relations | investigation_of_criminal_offences |
High-Risk? | Yes (Annex III. 1-a & 4-b) | Yes (Annex III. 6-a & 5-a) |
To show automation in determination of whether an AI system is
high-risk, and to show the usefulness of our analysis and AIRO’s
concepts, we created SHACL shapes, depicted in Listing 2,
representing two of the high-risk conditions defined in Annex III, and
then applied them over the use-cases. Annex III defines criteria where
systems are high-risk, and SHACL shapes are meant to fail when
constraints are not satisfied. Therefore, we modelled these SHACL shapes
to check where AI systems are not high-risk, that is - they
fail when a condition such as purpose being
BiometricIdentification
is met, with the annotation
assisting in identifying the source in Annex III-1.
Listing 2: Examples of SHACL shapes identifying high-risk AI Systems from Annex III of the AI Act
@prefix dash: <http://datashapes.org/dash#> .
@prefix sh: <http://www.w3.org/ns/shacl#> .
@prefix airo: <https://w3id.org/AIRO#> .
@prefix rdf: <http://www.w3.org/1999/02/22-rdf-syntax-ns#> .
:AnnexIII-1
a sh:NodeShape ;
sh:targetClass airo:AISystem ;
sh:message "High-Risk AI System as per AI Act Annex III-1"@en ;
sh:description "Biometric Identification of Natural Persons"@en ;
sh:not [
a sh:PropertyShape ;
sh:path airo:hasPurpose ;
sh:class airo:BiometricIdentification; ] .
:AnnexIII-6a
a sh:NodeShape ;
sh:targetClass airo:AISystem ;
sh:message "High-Risk AI System as per AI Act Annex III-6a"@en ;
sh:description "AI systems intended to be used by law enforcement..."
"... or the risk for potential victims of criminal offences;"@en ;
sh:not [ sh:and (
sh:property [
a sh:PropertyShape ;
sh:path airo:isUsedWithinDomain ;
sh:hasValue airo:law_enforcement ;
]
sh:property [
a sh:PropertyShape ;
sh:path airo:hasPurpose ;
# omitted (sh:or .. airo:AssessingRiskOfReoffending) here for brevity
sh:class airo:AssessingRiskOfReoffending ; ] ) ] .
We preferred SHACL since it is a standardised mechanism for expression validations, it always produces a Boolean output, and it can be annotated with documentation and messages. Also, SHACL has been demonstrated to be useful for legal compliance tasks where constraints can first ensure the necessary information is present and in the correct form, and then produce outputs linked to appropriate legal clauses [28].
Generating Technical Documentation
To demonstrate how AIRO assists with producing technical documentation as required by Art. 11 and described in Annex IV of the AI Act, we utilised SPARQL queries to retrieve the information regarding the two use-cases. The (summarised) results of this are shown in Table 4. Within the table, the “N/A” cells represents lack of information in the available sources regarding the related concept. For the sake of brevity, the rows with “N/A” values for both use-cases are excluded from the table.
In the future, we plan to demonstrate the application of AIRO in modelling multiple, different use-cases where comprehensive information about the AI system and its risks is publicly available.
Anx.IV. Required Information | Concept | Uber’s Real-time ID Check | VioGén system |
---|---|---|---|
1(a). System’s intended purpose | Purpose |
biometric_identification_of_drivers _to_decide_on_contract_termination | assessing_risk_of_gender_violence _recidivism determining_access_to_police _protection |
1(a). System’s developers | AIDeveloper |
uber | the_spanish_secretary_of_state_for _security |
1(d). Forms in which AI system is placed on the market or put into service | AISystemForm |
service | software |
2(e) & 3. Human oversight measures | HumanOversightControl |
manual_review | manual_modification_of_risk_score |
2(g). Discriminatory impacts of the system | Impact ImpactedArea |
unfair_dismissal_of_bame_drivers non-discrimination | lower_risk_scores_assigned _to_women_without_children non-discrimination |
3. Expected level of accuracy | AISystemAccuracy |
high | high |
3. Foreseeable unintended outcomes of the risk . Consequences of the risk | Consequence |
failed_to_identify_some_bame_ drivers | (1) victim_at_risk_remains _unprotected (2) risk_score_is_not_manually _modified_when_necessary |
3 & 4. Sources of the risk | RiskSource |
bias_in_algorithm_training | (1) poor_quality_of_input_data (2) N/A |
4. Risks associated with the AI system | Risk |
inaccuracy_in_identifying_bame _drivers | (1) inaccurate_predictions (2) automation_bias |
4. Harmful impacts of the risk | Impact |
unfair_dismissal_of_bame_drivers | (1&2) victim_being_assaulted |
4. Severity of impact | Severity |
N/A | critical |
4. Impacted stakeholders | AISubject |
uber_driver_of_bame_background | victim_of_gender_violence |
4. Impacted area | AreaOfImpact |
non-discrimination | safety_of_victim |
4. Risk management measures applied | Control |
manual_review | (1) manual_modification_of_risk _score (2) N/A |
Conclusion & Further Work
In this paper, we presented AIRO - an ontology for expressing risk of harm associated with AI systems based on the proposed EU AI Act and ISO 31000 family of standards. AIRO assists with expressing risk of AI systems as per the requirements of the AI Act, in a machine-readable, formal, and interoperable manner through use of semantic web technologies. We demonstrated the usefulness of AIRO in determination of high-risk AI systems and for generating the technical documentation based on use of SPARQL and SHACL by modelling two real-world use-cases from the AIAAIC repository.
Benefit to Stakeholders
AIRO assists organisations in maintaining risk information in a machine-readable and queryable forms. This enables automating the retrieval of information related to AI systems and their risks, which is necessary to create and maintain technical documentation as required by Art. 11. Furthermore, by assigning timestamp values to the machine-readable risk information expressed by AIRO, organisations can keep track of changes of risks, which is useful for implementation of the post-market monitoring system requirements referred to in Art. 61. Utilising AIRO for modelling AI incidents helps with classification, collation, and comparison of AI risks and impacts over time. This can be helpful in addressing the gaps exist between the ongoing AI regulation and standardisation activities and real-world AI incidents.
Further Work
In the future, the design of AIRO and the SHACL shapes represented for determination of high-risk AI systems will be revisited in the light of the amendments to the proposed AI Act. Our future investigations aim to extend AIRO to (i) represent known categories of AI incidents through their identification within incident reports, such as from the AIAAIC repository, (ii) provide the information required for creating incorporated documents within the technical documentation such as system architecture, datasheet, and the EU declaration of the conformity, (iii) express fundamental risk management concepts from the ISO 31000 family, which are essential for modelling AI risk and impact assessments, and (iv) express provenance of AI risk management activities, which is helpful in the AI Act conformity assessment process and implementation of post-market monitoring systems, by reusing the PROV Ontology 5.
We plan to demonstrate application of AIRO in sharing risk information between entities in the AI governance and value chain. Given the similarity and overlap between the AI Act’s risk and impact assessments with the GDPR’s Data Protection Impact Assessments (DPIA), we aim to investigate how the use of AIRO can provide a common point for the information management and investigations regarding risks and impacts associated with use of AI.
Acknowledgements
This project has received funding from the European Union’s Horizon 2020 research and innovation programme under the Marie Skłodowska-Curie grant agreement No 813497, as part of the ADAPT SFI Centre for Digital Media Technology is funded by Science Foundation Ireland through the SFI Research Centres Programme and is co-funded under the European Regional Development Fund (ERDF) through Grant#13/RC/2106_P2. Harshvardhan J. Pandit has received funding under the Irish Research Council Government of Ireland Postdoctoral Fellowship Grant#GOIPD/2020/790.
References
Prior Publication Attempts
This paper was published after 2 attempts. Before being accepted at this venue, it was submitted to: Annual Privacy Forum 2022