Annual Privacy Forum 2024
published:
by Harshvardhan J. Pandit
is about: Annual Privacy Forum
academic AI-Act conference consent GDPR
Introduction
The Annual Privacy Forum is an event organised by the ENISA (EU Commission's Network and Information Security Agency) and is an academic conference that invites talks related to privacy, data protection, cybersecurity, and associated topics. It is a prestigious event due to its close collaboration and involvement of policy makers as stakeholders, and it is typical to see several data protection authorities, standardisation experts, consumer organisations, and other stakeholders at the event. The event itself was organised across two days from 4th to 5th September, and the IPEN event was held on 3rd September before the conference (as is tradition).
The 2024 edition was held at Karlstad University in Karlstad, Sweden, and was co-organised by the EU Commission's DG CNECT - the Directorate-General for Communications Networks, Content and Technology (the section that looks after GDPR, AI Act, etc.) In total, there were (approx.) 62 submissions, of which 12 were accepted for presentation - giving us an acceptance rate of (approx.) 19.5%. The proceedings are available online. The next year, APF will (tentatively) be held at Goethe University in Frankfurt with the same approximate deadlines for submissions.
My involvement in the APF this year was to present two papers: Implementing ISO/IEC TS 27560: 2023 Consent Records and Receipts for GDPR and DGA where I was a lead author, and AI Cards: Towards an Applied Framework for Machine-Readable AI and Risk Documentation Inspired by the EU AI Act where I was a co-author. In addition, I also had the chance to 'expose' the audience to the preprint of a paper recently completed which compared the DPIA guidelines from all 30 EU/EEA DPAs and aligned them with the AI Act to show that GDPR is applicable in most cases. Below are my notes which I took during the event, including certain statements or resources which I found to be interesting.
I was quite pleased to attend the event as also in attendance was the EDPS, and members from the EU Parliament's legal team, ENISA, Data Protection Authorities from Germany (ULD), Poland, Italy, Spain, and Sweden. This meant that the work that I presented was visible to them - and indeed in conversations with them later all three papers mentioned by me were stated to be of interest.
Favourite Talks
My favourite talks at the event (not including my own, haha) were:
- Implications of age assurance on privacy and data protection: a systemic threat model - AEPD
This was a paper presented by the Spanish DPA on developing a systematic approach to understanding the threats of privacy and data protection. My favourite bits in this were that its a DPA following a really cool research approach to their compliance and enforcement knowledge, and that they extended the state of the art to create a new threat taxonomy. - Privacy Technologies in Practice: Incentives and Barriers - @LiinaKamm
This was a talk that nicely outlined the different PETs that can be utilised and what are the considerations that we should have when adopting them. It also included 'consent receipt' which I had presented in the previous day. - How to Drill Into Silos: Creating a Free-to-Use Dataset of Data Subject Access Packages
I liked this talk because it exposed the currently weak nature of Art.20 Data Portability compliance - namely not useful at all because of the unstructured mess that is returned. What I also learned was how Art.20 data is often also related to the other data e.g. Art.15 - which is something I hadn't thought about. The cool part of this work was the creation of a free/open dataset for requests by specific providers for use in research.
IPEN 2024-09-03
- theme: Human Oversight of Automated Decision-Making systems
- Introductory talk by @WojciechWiewiórowski
- we don't call them problems but call then challenges because they lead to solutions by the people who design and implement information systems
- automation in making important decisions about health, safety, and fundamental rights
- human in the loop does not mean everything is okay
- it will be pointless to scrutinise every single decision - instead we have to identify those decisions which need further introspection to understand what is happening or what must be understood
- Keynote: "Expectations vs. reality: What we expect human oversight to be and
what it really is" by @BenGreen
- paper: "The flaws of policies requiring human oversight of human algorithms" in Computer Law & Security review 2022
- Approaches to human oversight:
- restrict solely automated decisions - make human decision making mandatory e.g. GDPR
- require human discretion - human decision makers have ability to override the automated decision
- require meaningful human input - humans can override the system, understand the algorithm e.g. XAI, and human should not rely heavily on algorithms, such as in the AI Act
- Assumption of Human Oversight Policies
- people won't rely on automated systems
- people will follow the 'good' recommendations and override 'bad' recommendations - assuming people will know when to follow the recommendation and when to ignore/override recommendation
- explanations and interpretability improves human oversight - assuming that people will understand what action to take with the advice/explanation
- overseers will have agency to ignore the automated system - assuming that there is an ability to do so
- if we don't ensure the policies provide the benefits as expected, then we don't get the benefits and the system turns into an obfuscated automated system that has underlying issues
- Assumption 1:
- people including experts are susceptible to automated bias
- people defer to automated systems
- Assumption 2
- people are bad at judging the quality of outputs and determining when to override the outputs
- human judgements are typically incorrect and can introduce racial biases
- Assumption 3:
- explanations and interpretability do not improve human oversight, but instead up up increasing trust in algorithms regardless of the quality of the algorithm
- explanations likely to make people accept incorrect advice - which can cause people to be more dependent on the system
- algorithmic transparency reduce people's ability to detect and correct model errors
- frontline works face institiutional pressure to follow the recommendations of the system - and it might be more work for decision makers to ignore the recommendation e.g. there is paperwork
- human oversight policy from automated systems and their developers/providers to human operators
- implications: we need to think broadly how we are going to approach the design and implementations of automated systems
- lessons for regulating automated systems
- design and training can improve human oversight but cannot solve these issues - this is not a solution
- not using human oversight to justify harmful and flawed automated systems - many policies call for human oversight which legitimises use of problematic systems i.e. if a system is prone to errors and issues on its own, then it is also problematic to use with human oversight
- require pre-deployment testing of proposed human oversight mechanisms - to analyse and understand whether it can work with and produce decisions that we find acceptable
- shift accountability from overseers toward policymakers and developers
- Panel: "Human oversight in the GDPR and the AI Act" with @ClaesGranmar
@BenWagner moderated by @DanieleNardi
- Argument by @ClaesGranmar
- GDPR covers only one right - data protection and privacy whereas the AI Act covers all rights
- Law creates realities, which then become human standards and habits, and we don't realise it and take it for granted
- It is human tendency to push the liability to the system - even if it is an ineffective system someone must be liable and be held accountable from the legal perspective. This is why the AI Act addresses the providers and deployers.
- There are no good reasons for why we should not have rules for AI systems regarding health, safety, and fundamental rights
- GDPR is more ex-post-facto whereas AI Act is ex-ante in the sense that GDPR requires processing of personal data whereas the AI Act is a product safety regulation and treats the process of certifying and monitoring
- Argument by @BenWagner
- what is creating problems is the legal frameworks rather than institutions and policies
- Under the GDPR and other regulatory systems there is an impetus to claim that it isn't an automated system to escape the obligations
- The protections that are received should be the same whether its automated or manual. The presumption of automation should be present throughout the legal framework.
- This avoids problems with systems that seem to be manual but are actually automated - which we found in our research
- Moderated question: What do we do with what we have and the legal instruments that we have? @ClaesGranmar - the answer is proportionality of the law
- Audience question: got rejected at border control because of dark skin - whom to approach if the government is the one who is discriminating (in creation of AI systems) - moderator: go to ICO as this would be a case of using biometrics for decision making; but the human in the loop in this case works; not sure if there is a solution to the human in the loop
- Argument by @ClaesGranmar
- Panel: "Capacity Building" with @SarahSterz, @LeilaMethnani with moderator
@IsabelBarberá
- @SarahSterz - Center for Perspicuous Computing
- Art.14 AI Act says human oversight must be effective, but it doesn't state what is considered effective - creating a framework for when human oversight is effective
- working definition of human oversight: supervision of a system by at least one natural person typically with authority to influence its operations or effects
- framework:
- epistemic access: human should understand the situation and fundamental risks and can interact with the system to mitigate risks - understand system, how it works, outputs, etc.
- self-control - can decide path of action and follow through
- causal power - power to establish causal connection
- with these three things the human can mitigate fundamental risks reliably
- the first three are moral responsibility
- fitting intentions - has a fitting intention for their role i.e. the person wants to mitigate the risks
- factors to facilitate and inhibit human oversight - technican design, individual factors, environment
- two issues in AI Act that this work helps with:
- Art.14(4) lacks structure
- Art.14 does not provide definition of effectiveness
- @LeilaMethnani
- automated decision making used daily e.g. spotify playlists, spam detection and filtering
- High-risk makes the difference
- many questions about Art.14 - what is effective?
- It states "oversight measures" should match the risks and context - what about non-forseeable risks?
- AI sustems should be provided in a way that allows the overseer to understand its capabilities and limitations
- Human in the loop - RLHF is presented as human oversight - paper: Lindstrom, Adam Dahlgren et al "AI Alignment through Reinforcement Learning from Human Feedback?" arXiv
- Meaningful Human Control - track relevant reasons behind decisions an trace back to an individual along the chain
- Variable Automany for MHC (above) - involves transfer of control between human and machine - which aspects of autonomy can be adjusted? By whom? How? Continous or discrete? Why? Pre-emptive, corrective, or just because. When?
- The oversigh measures should match rhethe risks and context
- Paper: Methnani L. et al. 2021 Let me Take Over: Variable Autonomy for Meaningful Human Control in Frontiers Artificial Intelligence
- Efficient transfer of control is an open problem
- XAI is a double edged sword - different methods of explaining may lead to mailicious use of XAI
[ ]
Paper: AI Act for Working Programmers - https://doi.org/10.48550/arXiv.2408.01449
- @SarahSterz - Center for Perspicuous Computing
- Panel: "Power to the people! Creating conditions for more effective human
oversight" with @SimoneFischerHübner, @JahnaOtterbacher, @JohannLaux moderated
by @VitorBernardo
- argument by @SimoneWurster
- Humans overly trust AI, which is a problem because decision makers may lack awareness of AI risks. Therefore education to decision makers on AI limitations is important
[ ]
Alaqra et al. (2023) Structural and functional explanations for informing lay and expert users: the case of functional encryption - functional explanations for PETs needed for comprehensibility with complementing structural explanations for establishing trust. Multi-layered policy.- AI & Automation for usable privacy decisions: privacy permissions, privacy
preferences, consent, rejection – comparison. Manual, semi-automated,
fully automated. - Victor Morel IWPE
- GDPR issues for full automation except to reject consent
- semi-automated as privacy nudges have ethical issues
- argument by @JahnaOtterbacher
- Artificial Intelligence in Everyday Life: Educating the public through an open, distance-learning course - published paper 8 ECTS course involving explaining AI to someone younger and then seeing how the explanation changes after education
- modular oversight framework where users are developers - Modular Oversight Methodology: A framework to aid ethical alignment of algorithmic creations. Design Science (in press)
- argument by @SimoneWurster
APF Day 1 2024-09-03
5.1. My Paper: Implementing ISO/IEC TS 27560: 2023 Consent Records and Receipts for GDPR and DGA
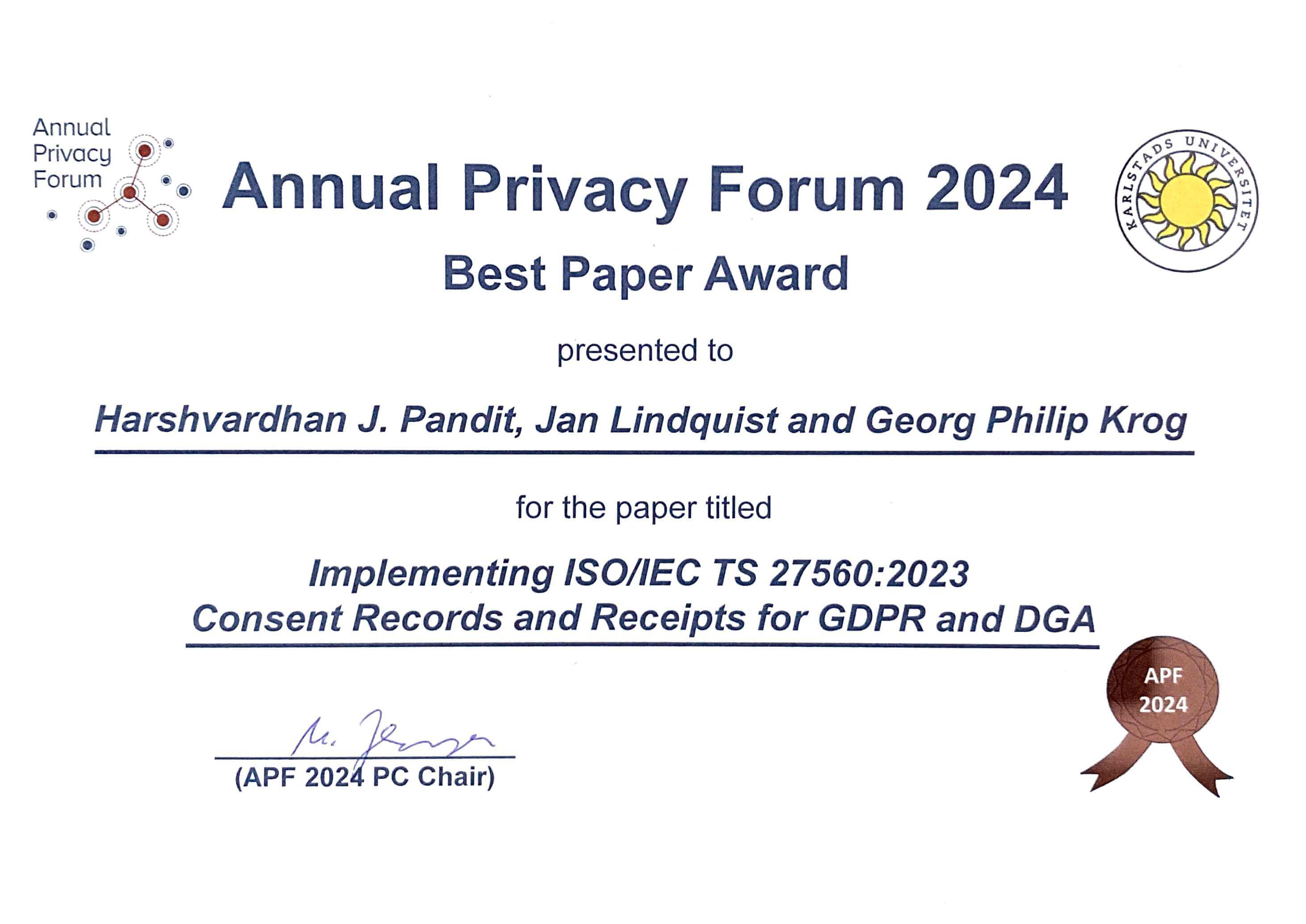
- This was the first paper presentation of the day - so starting the conference was a highlight for me as it means you have the attention of the entire audience.
- The presentation went smoothly where I presented for 20mins including together with my co-author Jan Lindquist, and then we have 5mins for the Q&A.
- The paper is available online as open access and the slides are available online as well.
5.2. Pay-or-Tracking Walls instead of Cookie Banners
- assess whether websites keep their privacy promise and offer pay options safeguarding privacy
- what do websites promise users who purchase the pay option?
[ ]
tracking ref. Karaj et al 2019, Mayer and Mitchell 2012- tracker - not essential third party service - requires consent due to ePD
- distinction between trackers and essential third party services
- discrepancy between promise and reality for cost-free decisions to refuse consent or opt out
[ ]
findings in related work: inappropriate tracking permissions in TCF consent string Morel et al, Tracking embedded in top publishers Mulle-Tribbensee et al 2024, Rasaii et al - no third parties embedded in multi-website provider[ ]
Empirical study: 341 websites from Morel et al 2023, visit website, extract promise - manual retrieval and go through first layer of dialogue, measure tracking using Ghostery Insights and checking tracker names and categories, check if the tracking starts before making the choice, then whether tracking starts after accept, and if tracking is still present after pay option- manually review the list to distinguish between tracker and third party essential service using Ghostery essential category, and using list by CNIL of privacy friendly site analytics services
- results: 292 websites identified after refinement, most from Germany (92%), category of website e.g. news, type of pay-wall - whether own pay option (36.6%) or multi-platform provider (63.4%)
- some technical problems using the pay option - e.g. option worked only 1-2 days after purchasing, or suggesting that accept tracking then log in
- tracking before and after making the choice
- 70 formulations for privacy promises e.g. without tracking and cookies, without ad tracking, etc.
- cluster them into "no tracking" (65.4%) and "no ad tracking" (34.6%)
- then whether tracker was detected in the option, and then conclude whether promise is kept (67.1%) or not kept (32.9%)
- 80% of websites still embedded tracking in pay option: 1 or 2 trackers
- no difference across country or industry
- difference in whether website used own pay option or multi-provider - 60.7% own pay did not keep promise, multi-website break promise 16.8%
- potential reason of this - multi-website provider use daily crawls to detect and control the trackers
- conclusion: 80% of websites could meet privacy commitments by removing just 1 or 2 trackers, ongoing tracker control or continous checking helps perform better - multi-website provider
- regulatory suggestions: support/mandate ongoing tracker detection, and support/initiate standardised privacy label as external validation
- reported to multi-website provider representing 185 websites, reporting BVDW german association for digital economy which is DE advertising federation which resulted in asking individual websites
5.4. Implications of age assurance on privacy and data protection: a systemic threat model - AEPD
- age assurance: determine age or age range with different levels of conditions or certainty e.g. online age gate
- age estimation: probabilitic, age verification: deterministic
- purpose is guaranteeing children's protection and a safer internet - but also raises concerns about privacy and data protection
- CRIA - child rights impact assessment; protect from consumer, contact, conduct, and content risks while balancing rights and freedoms through the DPIA (CRIA is about child's risks, DPIA is about rights and freedoms)
- privacy threat modelling frameworks provide a structured approach to identifying and assessing privacy threats
- contributions:
- systematic analysis of age assurance solutions
- proposed threat models to identify threats based on GDPR and rights
- discuss threat models to offer practical and evidence based guidance
- literature review: papers, patents, technical documentation - 8 solutions and
4 prototypes
- 3 architectures:
- direct interaction between user and provider who ensures age without involving specialised providers
- tokenised approaches relying on third party providres who can be acting as a proxy or as synchronous agents (user gets token to provide)
- 3 architectures:
- LINDDUN privacy threat model framework is valuable to support effective DPIA
- proposed LIINE3DU framework - more details will be published by AEPD later
- Linking, Identifying, Inaccuracy, Non-repudiation, Exclusion, Detecting (LIINED) + Data Breach, Data Disclosure, Unawareness and Unintervenability
- LIINE3 = data subject rights and freedoms
- DU - organisation's risk management and data processing design - Not GDPR compliant if applicable
- This work is focused only on privacy and data protection threats
- identified 18 different threats
- created recommendations for providers based on common mistakes and vulnerabilities
- common vulnerabilities:
- use of unique identifiers
- used date of birth or specific age even when not required
- exchange of tokens used excess of information
- data retained for longer than necessary
- involvment of children in assurnace processes - but only users above the age threshold should be involved in age assurance
- incentives for fraud
- existence of only alternative to assure age, often complex, and difficult to use
- concerns for estimation based approaches
- concerns for verification based approaches
- some threats cannot be avoided with available architectures
- industry and researchers should explore how to:
- perform age assurance process on users' local devices
- consider decentralised and user-empowering solutions such as EUDI wallets
- rely on selective disclosure and zero-knowledge proofs
- regulators, policymakers, and standards bodies to focus on harmonising terms, nomenclature, technical solutions, and co-operating with industry and NGOs to establish global certification schemes, codes of conduct, and regular auditing
5.5. Data Governance and Neutral Data Intermediation: Legal Properties and Potential Semantic Constraints
- Emanuela Podda Uni. Milano
[ ]
Ref. Creating value and protecting data - Vial 2023, Approach to meet compliance with regulatory and legal provisions - Otto 2011[ ]
Normative: rule-making approach multi-layered and multi-regulatory legal system - Viljoen 2021.[ ]
Co-regulatory approach: accountability - Black 2001[ ]
Role and Resonsibilities: liability allocation - Floridi 2018
- Data Governance is composed of different and emergent components, which in
turn are composed of other interactinos taking place at different levels
- macro - overaching legal system
- meso - corporate
- micro - individual
- types of intermediation
- access to meta-data vs access to raw-level data
- active or passive
- undertaking data sharing - active intermediation
- facilitating data sharing - passive intermediation - has no access to data
- intermediation must be provided by a separate legal entity - which is a semantic constraint
- Rec.33 neutrality is a key element in enhancing trust - but is this enough?
- cloud federation scenario that promotes rebalancing between cetralised data in cloud infrastructure and distributed processed at edge
- proposed future work - to create a regulation combining DGA with DSA
5.6. Threat Modelling
[ ]
STRIDE[ ]
MITRE ATTACK[ ]
LINDDUN[ ]
MITRE PANOPTICON- Threat modelling to structured DPIA
- Bringing privacy back into engineering
5.7. Another Data Dilemma in Smart Cities: the GDPR’s Joint Controllership Tightrope within Public-Private Collaborations
- Barbara Lazarotto and Pablo Rodrigo Trigo Kramcsak - VUB
- GDPR allows multiple legal bases based on wording of Art.6 "at least one of the following applies..."
- multiple legal basis is a problem - lack of clarity on how this works
- my comment during Q&A: multiple legal bases does not mean we can combine any two legal bases and use them - some of them lead to nonsense like using Legitimate Interest which is purely opt-out and Consent which is purely opt-in together which creates a Schrodinger's legal basis which both is active and isn't depending on whether the data subject has consented or objected. Instead, multiple legal bases allow for Member States to create specific legislations e.g. for public bodies to act in official capacity or for private bodies to be required to do something. However, for private bodies who have relationships with data subjects, contract would be the legal basis in addition to the legal obligation or the official authority of the controller being used in a joint controller relationship.
5.8. The lawfulness of re-identification under data protection law
- Teodora Curelariu and Alexandre Lodie
- why research in re-identification is important for improving privacy and data protection?
- doctrine:
- objective: anonymised data where reasonably likely means to re-identify are not considered
[ ]
relative: reasonably likely means used to re-identify are considered - prevails in EU Law C-582/14 Breyer - IP Address are personal data[ ]
Case T-557/20 SRB vs EDPS - personal data are not always personal based on whether entity is likely to re-identify; which means pseudonymised data can be considered as anonymised data based on ability to re-identify
- implications: entities that cannot re-identify can freely share the data, thus
exposing personal data
- if data is considered anonymous, then data can be freely transferred across EU borders
- can re-identification for research purposes be lawful under GDPR?
[ ]
Norwegian DPA: entity performing re-identification must be considered controller- researchers re-identifying data are controllers and must have a legal basis e.g. to find security vulnerabilities
- scientific purpose by itself is not a legal basis
[ ]
CNIL guidelines on data processed for scientific processes which states there are strict legal basis: consent from subject, public interest, or legitimate interest[ ]
UK DPA 2018 prohibits re-identification without consent except for research- Rec.45 states countries should have specific rules for research purposes
My Paper: AI Cards: Towards an Applied Framework for Machine-Readable AI and Risk Documentation Inspired by the EU AI Act
- This paper was led by Delaram, who is doing her PhD on this topic. It was a collaboration with the EU Commission's Joint Research Centre (JRC) - and I clarified that the views expressed in the paper are those of the researchers and do not reflect the views of the Commission.
- The paper is available online as Open Access and the slides are avialable online as well.
- The paper describes creation of a human-intended summarised visual overview of the risk management documentation based on the AI Act and the available ISO standards at that point in time.
- The work generated a lot of interest due to the AI Act being a core topic at the event, and because of the practicality of the work.
APF Day 2 2024-09-04
6.1. Keynote: Privacy and Identity - @JaapHenkHogman
- eIDAS 2.0
- EUDI
- app on smartphone
- used by Member states - Architecture Reference Framework 1.40 May 2024
- attributes, certificates, documents: a personal data store
- Apple/Google issuing IDs is a fundamental threat to the social structure of governance
- attribute attestations - claims based authentication
- issuer I claims that Person P has Value V for Attribute A
- selective disclosure - only reveal required attributes
- self-sovereignty: decide what attestations to get, and from whom
- decouple getting and using an attribute (issuer unlinkability) - present issuer from learning when and where you use an attribute - significant issue in social logins
- decouple successive uses of an attribute - multi-show unlinkability - prevent profiling be relying parties using attestation signature as persistent identifier
- still guarentee security of attributes - increased by binding to a trusted hardware element
- problem 1: attribute attestations in eIDAS 2.0 are lame
- a set of signed salted hashes
- can do selective disclosure
- issue: decouple getting and using an attribute - issuer knows signature, signature is revealed to relying party ; when relying parties collude with issuers, users can be profiled
- issue: decouple selective uses of an attribute
- solution: issue many attestions with different salts
- better to use attribute based credentials based on zero knowledge proofs which
don't reveal the signature abut prove you have it - which gives true
unlinkability between issuer and relying party
- issue: not using "state approved" cryptographic primitives
- issue: not implemented in current secure trusted hardware components - which is important because we want to make sure the credentials cannot be copied to another device which requires hardware level support
- more issues
- assumes people have smartphones that can run the wallet - which creates inclusion problems and creates single point of failure
- risk of over identification - authenticated attributes supply will lead to increased demand where these are asked for more than is necessary and mandatory acceptance on large platforms
- under-representation - some entities decide what attributes are designed in the system and what values are available e.g. age ranges or gender
- privacy: requirement in the regulation that you should be able to revoke attributes and wallet fast - which means the attributes have to be checked (for validity) often
- wallet issuer will know when and where the wallet was used
- wallet also proposed for digital euro
- mixing high and low security use cases
- NL has bonus cards and passports - which have different security levels
- but the regulation asserts that both these should be part of the same wallet
- people may not understand that the device is used for low and high security contexts which can create issues such as phishing attacks
- preventing over-authentication
- encode what attributes the relying part is requesting should be included in the request to prevent over-authentication
- e.g. I need to verify someone is an adult, give me DOB, but instead the attribute that should be allowed is the is-adult
- issuer can specify a disclosure policy - but this is simplistic - no discloure, disclosure, and a group disclosure - which assumes the issuer can see all the uses of its attributes
- general observations
- technical specifications developed without oversight or academic or civil society participation - which is an issue as the technical specifications determine real security/privacy properties
- problem with standardisation - costs time and money, influence depends on level of participation, more stakeholders e.g. NGOs should be involved but they don't have resources to do this
- consultation open till SEP-09 on implementing regulations
- don't make vague implementing regulations, but create one clearly defined standard and make that mandatory
6.2. Privacy Technologies in Practice: Incentives and Barriers - @LiinaKamm
- Cybernetica is a SME in Estonia that is research focused
- architects of the e-Estonia ecosystem which is the interoperable database and system used by Estonia
[ ]
PETs categorisations (add to DPV)- data protection during analysis
- pseudonymisation
- anonymisation
- restricted query interfaces
- analyst sandboxes
- differential privacy
- federated learning
- data synthesis
- trusted execution environments
- homomorphic cryptography
- secure multi-party computation
[ ]
anonymous communication: secure messaging, mixnets, onion routing[ ]
transparency: documentation, logging, notification of stakeholders, layered privacy notices, just-in-time notices, consent receipts- intervenability: privacy and data processing panels and self service, dynamic consent management
- privacy engineering in system lifecycle: in new systems, privacy engineering and PETs should already be incorporated at problem statement and requirements stage, and in existing systems it should be added during system redesigns
- what motivates use of PETs: efficiency, regulation, liability, emergency, we care about privacy
- US Census bureau switched to using differential privacy since 2020
- Scottish Govt. wants to create a natinoal data exchange platform that requires using PETs like multi-party computation - Challenge 10.4, deadline SEP-10
- PET concept and roadmap for e-Government in Estonia
- in beginning of 2023, Estonia conducted a research project that explores if we already have interoperability, how do we enhance it with PETs
- how could the Estonian govt. take up PETs and which fields
- interviews with 18 state agents
- prerequisites for uptake of PETs
- increase data quality
- cleaning datasets
- creating infrastructure for processing big data
- clarifying rules for data management and handling during crisis or emergency
- raise awareness of privacy and PETs
- creating a smart customer, teaching how to choose the right PET
- secure data spaces e.g. proving attributes and/or properties: e.g. proving age, or to get the subsidy based on distance driven
6.3. 10 considerations on the use of synthetic data - Giuseppe D'Acquisto
- Garante / Italian DPA
- synthetic data are mentioned in Art.10 of the AI Act regarding mitigation of biased outcomes and in Art.59 as enabler for further processing
- in US, mentioned in the executive order as a PET
- synthetic data may create "adverse selection"
- training synthetic data generators is a processing of personal data; which means it needs an appropriate legal basis under the GDPR - even including the assessment of the legitimate interest where relevant; another factor is the context of use - e.g. if the synthetic data is to be used for scientific research the conditions are possibly less stringent. This step should not be skipped by Controllers.
- synthetic data can be so well generated that they are "true personal data" - which means the synthetic data ends up reproduced as personal data e.g. a facial generation or generating a phoen number. And therefore this is also the processing of personal data. The nature of personal data does not depend on the artificiality of generation, but on the nature and implication of that data. If synthetic data has a low percentage of some of it being personal data, then it may still be an issue if the corups is billions of samples
- individual's rights must be preserved with synthetic data - primarily the right to object and to opt out ; but also to consider that using PETs like differential privacy can end up with private but useless data
- synthetic data capture well a median world, but they are not suited for new discoveries e.g. unforseen new personal data. If there is a strong outlier in the real world then it would not be reproduced in synthetic data.
- synthetic data can create model collapse - e.g. if there is an inference created based on synthetic data, and then this inference is used for other inferencing, then the iterations increasingly become conformative and do not reflect the reality as the distribution is increasingly normalised
- synthetic data generation is a transformation of personal data - whether these transformed data are personal or not does not depend on what the output looks but on how much original information is kept in the transformation.
- its not difficult to use synthetic data in a malicious way, but it is difficult to discover wrongdoers. This requires more regulatory oversight and attention.
- its all about regulating stochasticity - the issue is not new about whether we need more complex mathematical model for simulation or do we resort to synthetic data in a simple sense ; there is a risk of bias and discrimination
- the use of synthetic data does not mean prohibiting the use of personal data, but extracting the value
- "If you torture the data long enough, it will confess to anything" - Ronald Coase
- Garante is organising G7 meeting of DPAs and synethetic data, and some output or guidance is expected from the event. Synthetic data is also on the agenda of the EDPB and ENISA.
6.4. Panel: Capacity Building
- moderator: Marit Hansen (ULD)
- panelist: Christoffer Karsberg (MSB/NCC-SE)
- panelist: Simone Fischer-Hübner (CyberCampus/CyberNode Sweden)
- panelist: Caroline Olstedt Carlström (Forum för Dataskydd/Cirio)
- panelist: Magnus Bergström (IMY)
6.5. How to Drill Into Silos: Creating a Free-to-Use Dataset of Data Subject Access Packages
- DSAR is difficult
- E.g. Facebook DSAR request - received a zip file which contains a folder structure with folders and files
- in practice resulting formats of DSARs as per A.15 and A.20 are similar - i.e. Subject Access Request Package (SARP) (electronic copy of personal data)
- there is a lack of SARP datasets which limits research
- Contribution 1: initial SARP dataset
- goals:
- public and free to use dataset of SARPS
- machine-readable data
- detailed data
- realisitic data
- controlled data
- two dimentional analysis of dataset - no time in this study
- method to create dataset
- 1 and 3 should be research only accounts
- 2 and 5 have provided data - since we cannot know observed or inferred data
- 4 is from targeting gatekeepers from DMA
- initial dataset
- two subjects, five controllers (Google, Amazon, Facebook, Apple, LinkedIn)
- LinkedIn provides two SARPs for each request: first within minutes, and another one within hours
- Apple failed in first try in one case
- findings
- Amazon gives ads shown only for Amazon Music but not for Prime Videos
- Amazon - Information on targeting audience is only given to Subject A
- Contribution 2: creating SARP
- selection of controllers
- create account
- usage period
- access request
- pre-processing
- publication
- issues such as authentication error lead to repeating steps
- SARPs enable
- privacy dashboards - cross-controller visualisation of SARPs
- Mapping SARPs towards data portability
6.6. Access Your Data… If You Can: An Analysis of Dark Patterns Against the Right of Access on Popular Websites
- @AlexanderLobel
- Right of Access
- DSAR: Do operators use the control they have over their own websites to tire users from exercising the right?
- Using dark patterns
- Analysed Top 500 Tranco list, inclusion criteria = 166 websites
- created independent open coding based on common search protocol and created supporting screencasts to find and submit an access request
- recorded feelings on whether they felt this was a dark pattern
- findings: analysis of request mechanisms, whether information could be found